Applied Mathematics Group
Advancing FASSt-Simulation: A Novel Computational Framework for Model-Measurement Integration for Climate Prediction
Atmospheric observing systems are critical experimental platforms for improving process understanding of aerosol-cloud-climate interactions and land-surface feedbacks that exert strong control over emergent weather and climate patterns, and extensive networks of Earth observing systems have been deployed over the Earth’s surface, atmosphere, oceans, and in space. The planning and operational deployment of instrumentation at new observing sites is expensive and time-consuming, and it is important to ensure instrument selection and siting are guided by scientific expectations about the expected value of information provided by each design choice.
As part of Brookhaven’s Four-Dimensional Atmospheric Sensing and Simulation (FASSt) initiative, we are developing a mathematical and computational framework for optimal observing system design based on the principles of Bayesian optimal experimental design. The approach begins by calibrating Earth system models to existing observational data, using a combination of ensemble simulation, statistical/machine learning emulation, and Bayesian inference. The result is a probabilistic representation of present-day uncertainty about the Earth system. Using observing system simulation experiments (OSSEs), we simulate random realizations of potential measurements that might be collected under different instrumentation and siting design choices. We repeat the uncertainty quantification process on each potential experimental outcome and use the results to identify the observing system designs that are expected to most reduce uncertainties in model predictions.
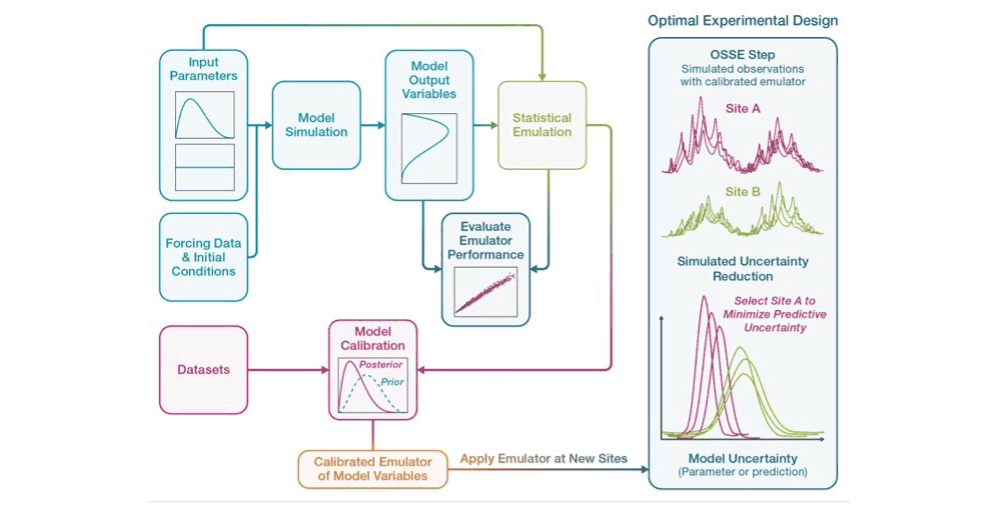
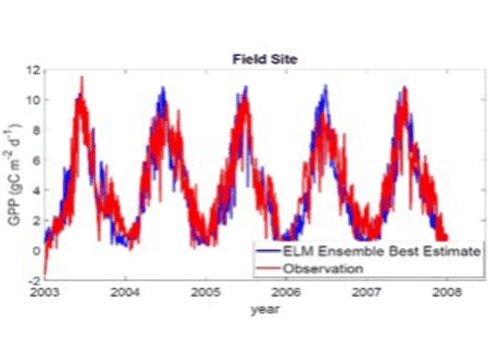
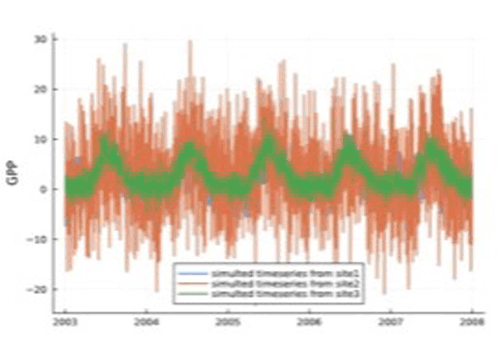