Applied Mathematics Group
Multifaceted Mathematics for Predictive Digital Twins (M2dt)
Digital twins are virtual computational representations of real-world systems that integrate measurements and other information to improve prediction and control in those systems. In the M2dt project, we are building and applying digital twins for two applications: the self-assembly of thin polymer films relevant to semiconductor and battery design and ice shelf-ocean cavities that are trigger points for sea level rise.
In this multi-institutional project led by the University of Texas at Austin, Brookhaven’s research on the first theme is conducted in collaboration with Brookhaven’s Center for Functional Nanomaterials (CFN), in coordination with the National Synchrotron Light Source II (NSLS-II), whose X-ray scattering measurements are used to characterize material properties. The material design problem involves block copolymer (BCP) systems consisting of two or more polymer types that naturally form patterns within a thin film. The geometric structure of these patterns, and the material properties, such as its conductivity or wetting characteristics, depend on the conditions under which the block copolymer forms, e.g., its temperature profile or patterning on the substrate underneath the film.
The mathematical challenges involve developing a digital twin of this system and using it to virtually evaluate many possible experimental conditions to determine how to drive the BCP formation process toward a desired target structure. We have developed a GPU-accelerated partial differential equation (PDE) reduced model of BCP dynamics, along with statistical and machine learning surrogate models of the PDE system, in a hierarchy of digital twins that predict BCP system behavior at different levels of fidelity and computational expense. We are applying Bayesian computer model calibration techniques to quantify uncertainties in the system dynamics. These, in turn, will be used in an optimal experimental design (OED) framework to select experiments to be performed that are maximally informative about how to improve the control BCP self-assembly toward a target. This forms a closed loop where predictive digital twins are used to control the material synthesis process, which, in turn, refines and improves the digital twins themselves.
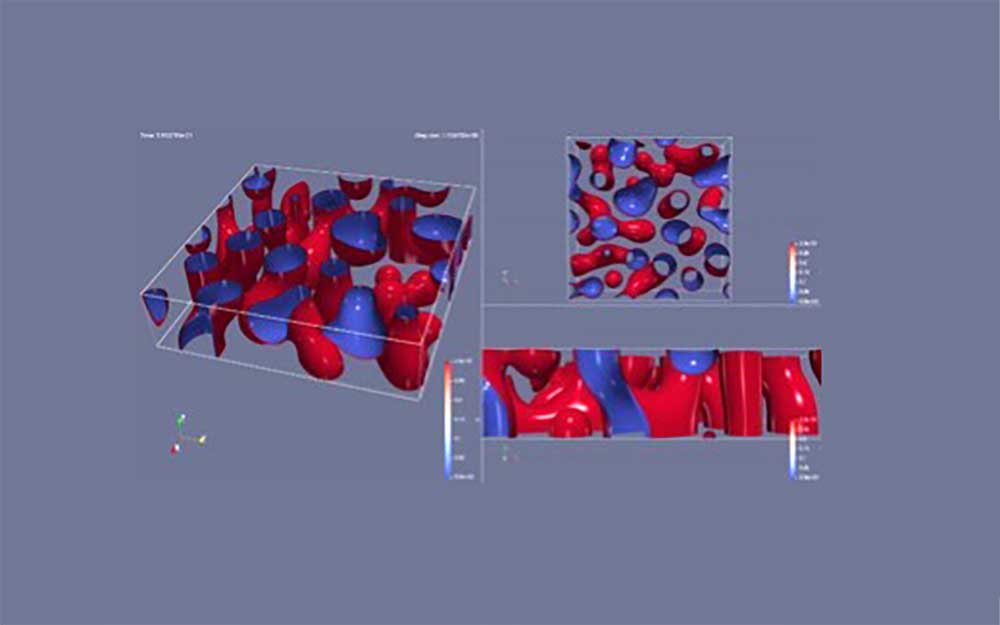