

Multi-scale
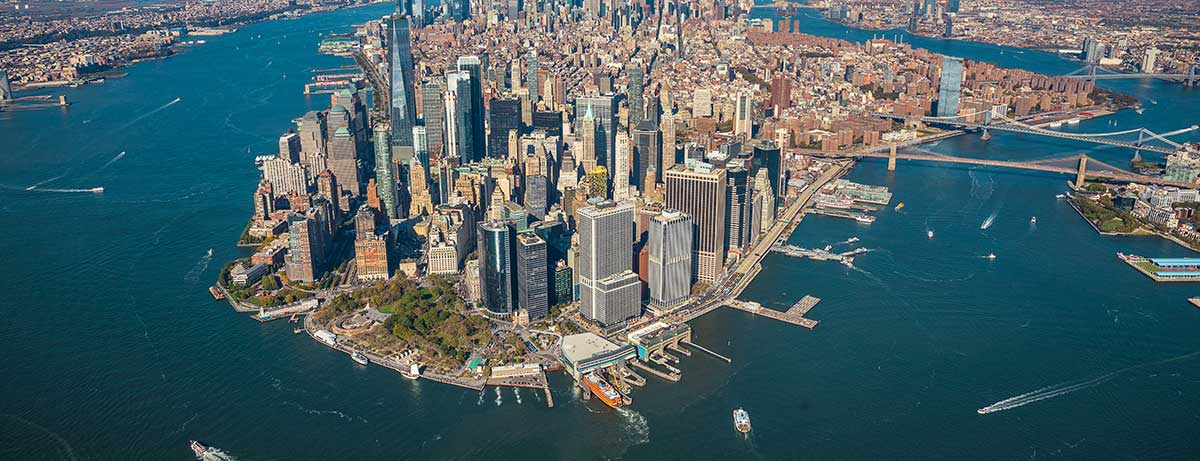
Developed urban and coastal areas are characterized by highly heterogeneous energy sources and landscapes, amplified micro-climate states (e.g., heat waves), and vulnerability to extreme weather and rising sea levels. Cities are rarely built on flat, homogeneous terrain, thus requiring consideration of topographic effects and local circulations. Many cities lie on coastlines and thus land-sea interactions must be considered (e.g., sea breeze circulations).
For example, the Northeastern megalopolis, stretching from Boston to Washington, contains more than 50 million people and represents 20 percent of the country’s gross domestic product. From an energy viewpoint, these areas have fluctuating energy demands and flows (exchanges) with the surrounding environment.
In addition to urban areas, renewable energy facilities need high temporal and spatial resolution predictions of wind and cloud conditions to optimize their energy output and its distribution through the national energy grid.
Such needs pose new challenges, perhaps greater than conventional weather and climate forecasting, in areas of both atmospheric (physical) and computational sciences. For example, (1) the necessity of forecasting extreme events, at high-resolutions, and over multiple time horizons calls for better representation of unresolved subgrid processes, especially cloud microphysics, radiative transfer, planetary boundary layer, turbulence, and atmosphere-surface interactions. (2) Development of accurate physical models also requires high-resolution measurements and their assimilation into physical models. (3) Both high resolution measurements and high-resolution physical modeling generate an explosive growth of data characterized by large volume, variety, velocity, and veracity.
The so-called big data science is causing revolutionary changes in many areas including science and technology. Data-driven modeling based on advanced machine learning (ML) has emerged as a hot topic in various fields. Limited studies have demonstrated that recent advances in data-driven models can be effectively used to improve short-term nowcasting, develop ML emulator for physical models or process parameterizations, and enhance/accelerate data assimilation. CMAS scientists in collaboration with other institutions, are addressing these challenges by capitalizing on the great advances in physical models, machine learning, and measurements.