Applied Mathematics Group
Multifaceted Mathematics for Predictive Digital Twins (M2dt)
This project is developing rigorous theoretical foundations for effective reduction of scientific data and implementing practical algorithms that can significantly reduce the data generated by complex experimental or computational systems. The overarching principle is to focus on the scientific objectives of data and on faithful retention of the quantities of interest (QoI) that pertain to these objectives while condensing the rest to a minimum. By taking such an objective-driven approach, we aim to achieve optimal data reduction with respect to scientific QoI, outperforming general-purpose data reduction techniques by a significant margin.
The project activities are grouped into three Research Thrusts. Research Thrust 1 explores and develops novel strategies that incorporate semantics and invariances to achieve transformational gains in scientific data reduction. We will leverage the group-theoretic invariances of data from scientific instruments and sources for data reduction in scientific workflows. Research Thrust 2 focuses on a Bayesian objective-driven data reduction framework. The framework will be used to develop objective-driven optimal data reduction strategies that minimize any expected adverse impact of data reduction on QoI in the presence of uncertainty. The framework will enable informed trade-offs between compression rate, resource utilization, speed, and impact on QoI. Research Thrust 3 is extending the foundational research in Thrusts 1 and 2 to a compositional setting to develop effective data reduction strategies for general scientific workflows.
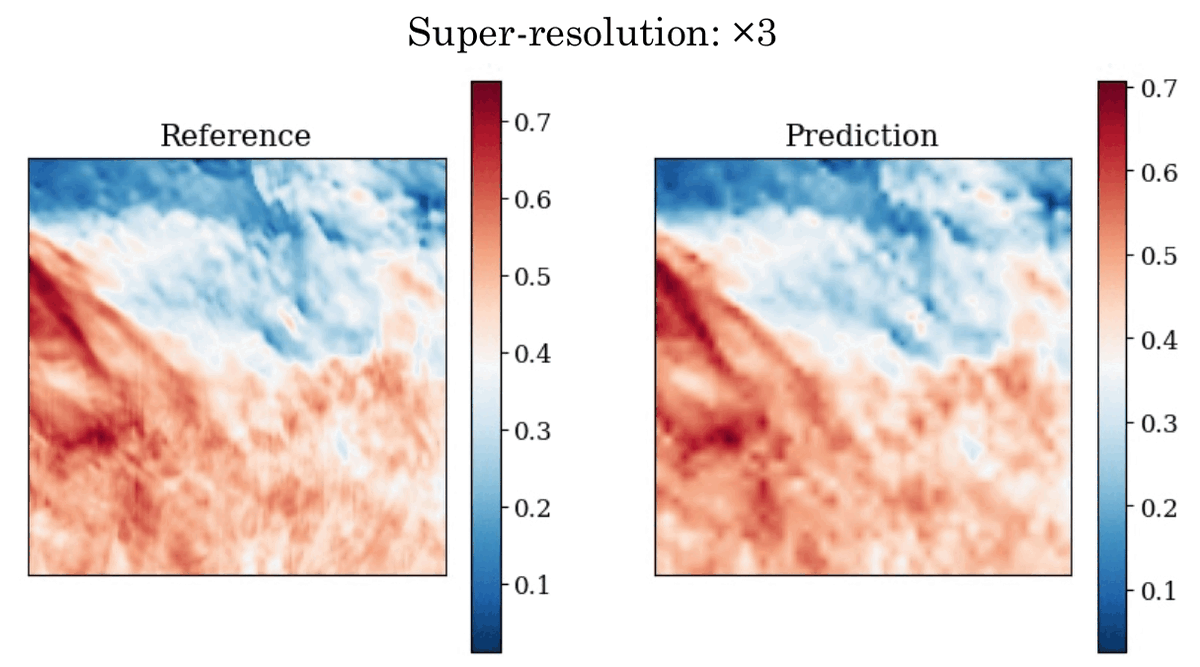