Applied Mathematics Group
Robust, Adaptive Decision-making via Impact-oriented, Uncertainty-aware Models (RADIUM)
Led by Brookhaven Lab with partners Los Alamos and Lawrence Berkeley national laboratories, the RADIUM project is developing a computational framework for optimal decision-making under uncertainty subject to the constraints of: 1) limited existing data and a narrow ability (cost, time, etc.) to acquire new data, 2) finite computing resources allocated optimally toward achieving specific goal(s), and 3) confined time frames to generate insight on which to base a decision. With this framework and accompanying computational tools, decision-makers will be able to evaluate not only the likely outcome of proposed actions, but also quantify uncertainty. By leveraging the highly performant software frameworks developed under the Exascale Computing Project (namely, the AMReX and ExaLearn Co-Design Centers), this project will focus on the calibration and integration of heterogeneous data sources and models. The tools developed by this multi-laboratory effort will be applicable to evaluations of mitigation procedures related to global epidemics, as well as other areas where agent-based models (ABMs) are used.
Especially, we aim to develop a computational framework for epidemiological modeling that is inherently uncertainty-aware and designed to facilitate optimal and robust decision-making in the presence of immense model and multimodal dataset uncertainties and subject to resource constraints. The framework also will enable rigorous quantification of uncertainties in epidemic predictions, offer accurate assessment of the expected efficacy of potential containment strategies and their prioritization, and aid in designing dynamic and intelligent acquisition strategies for data streams to improve modeling and decision-making. The RADIUM framework will enable automated model calibration to generate accurate predictions of future pandemic trajectories and allow users to design robust competence-aware mitigation/containment policies for a pandemic amid uncertainties, leading to the design of intelligent data acquisition strategies for reducing key uncertainties that can improve decision-making.
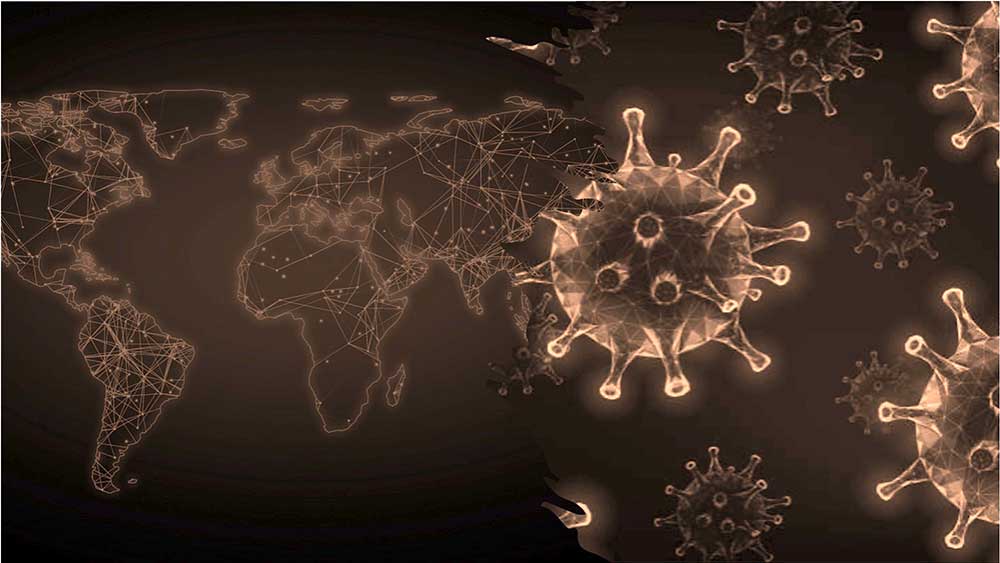