Faster X-ray Nanotomography Using Machine Learning
Scientists at NSLS-II enable users to study changes in materials faster than ever before
May 17, 2023
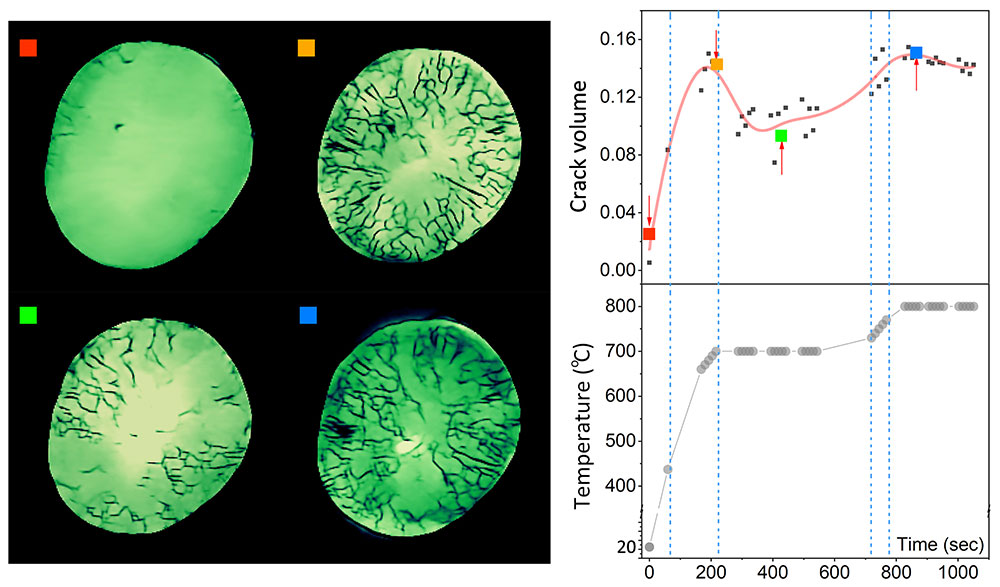
Figure showing the different stages of cracks in a battery particle during continuous heating. The left shows the cross section of the particle at four different times, while the right shows a graph of the crack volume at these four times
What do battery cathodes, molten salts, and atomic layer deposition have in common? To study the changes, growth, or chemical reactions in these materials, researchers must study them as they change. One tool for studying the internal dynamics of materials is x-ray computed tomography (CT). However, the time required to acquire a CT scan must be shorter than the timescale at which changes occur inside the material. The obvious solution of using a higher intensity x-ray beam can lead to parasitic reactions and damage to the sample, reducing the quality of the measurement.
To address this challenge, a team of researchers at the National Synchrotron Light Source II (NSLS-II) developed an image processing method based on machine learning that significantly reduces both data acquisition time and x-ray dose. Using the Full Field X-ray Imaging (FXI) beamline, the team was able to reduce the acquisition time to less than 10 seconds with a pixel resolution of less than 50 nanometers (nm) in a transmission x-ray microscope. FXI offers advanced capabilities for studying the morphology and oxidation states of dynamic systems in 2D and 3D with 30 nm resolution. The beamline is specialized in in-situ and operando studies of energy storage devices, as well as environmental, biological, and materials science samples. ALL NSLS-II beamlines—including FXI—are available free of charge to academic users through the NSLS-II user program.
In their study, the researchers demonstrated that their method outperforms conventional methods such as filtered-back projection, maximum likelihood, and model-based “maximum a posteriori” probability by up to a factor of two, depending on the reconstruction method used. The new algorithm is based on a supervised deep learning neural network using residual in residual dense block (RRDB) to correct image artifacts induced by fast data collection. The performance of a supervised neural network is highly dependent on the quality of the training dataset. To achieve better performance, simulations were used to generate a bank of blurred 3D reconstruction images based on the derived mathematics that characterize the blurring and noisy features originated in fast tomography at beamline. The model incorporates several loss functions, including mean absolute error, mean squared error, and visual geometry group VGG feature loss.
The team applied this machine learning algorithm to study the dynamic morphology changes in a lithium-ion battery cathode when the cathode was heated at a rate of 50°C per minute. The study showed that thermal annealing at this rate allowed cracks to heal themselves.
The researchers conclude that this new method can be applied to several other fields of study using similar conditions. This is supported by the fact that their new method can be applied to all other tomography measurements using different signals. This would even allow its use in medical imaging, where a reduction in acquisition time and dose would be beneficial.
The team for this study consisted of Jiayong Zhang, Wah-Keat Lee, and Mingyuan Ge.
The work was supported by the U.S. Department of Energy.
The National Synchrotron Light Source II (NSLS-II) is a U.S. Department of Energy (DOE) Office of Science user facility located a DOE's Brookhaven National Laboratory.
Brookhaven National Laboratory is supported by the Office of Science of the U.S. Department of Energy. The Office of Science is the single largest supporter of basic research in the physical sciences in the United States and is working to address some of the most pressing challenges of our time. For more information, please visit science.energy.gov.
Follow @BrookhavenLab on Twitter or find us on Facebook.
2023-21220 | INT/EXT | Newsroom