Predicting X-ray Absorption Spectra from Graphs
By representing the structures of molecules as graphs, scientists built a machine learning model that can rapidly predict how atoms absorb x-rays—a process that is key to understanding the structural and electronic properties of materials and molecules
July 16, 2020
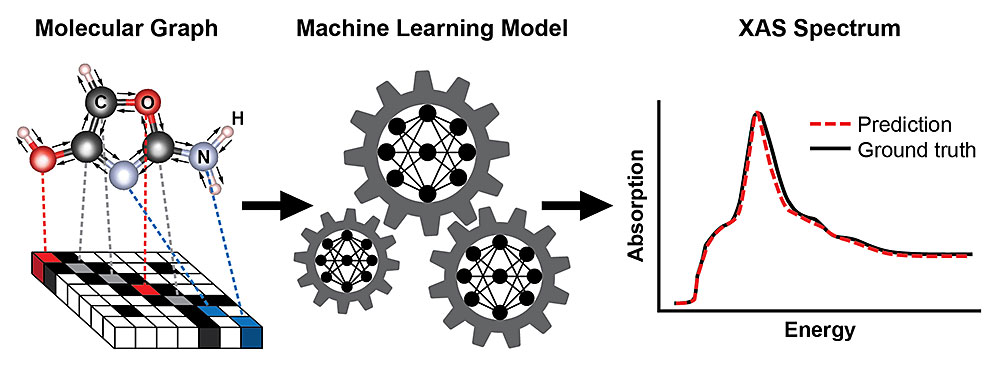
A schematic showing the steps for training a machine learning model to predict an x-ray absorption (XAS) spectrum based on the known structure of a molecule. The molecule's structure is represented as a graph, with atoms as nodes and chemical bonds as edges. This representation captures the connectivity of atoms—here, carbon (C), oxygen (O), nitrogen (N), and hydrogen (H)—and the type and length of the chemical bonds connecting them. The resulting XAS spectrum contains rich information about the local chemical environment of absorbing atoms, such as their symmetry and the number of neighboring atoms.
X-ray absorption spectroscopy (XAS) is a popular characterization technique for probing the local atomic structure and electronic properties of materials and molecules. Because atoms of each element absorb x-rays at characteristic energies, XAS is well suited for mapping out the spatial distribution of elements in a sample. Typically, scientists perform XAS experiments at synchrotron light sources—such as the National Synchrotron Light Source II (NSLS-II)—because they provide very bright, tunable x-rays. By measuring the absorbance in a sample at varying x-ray energies, scientists can generate a plot called an x-ray absorption spectrum.
“XAS is a key capability for users at Brookhaven National Laboratory’s NSLS-II and the Center for Functional Nanomaterials (CFN), both U.S. Department of Energy (DOE) Office of Science User Facilities that are open to the scientific research community,” said Deyu Lu, a physicist in the CFN Theory and Computation Group. “With the right analysis tools, XAS can provide tremendous insights in nanoscience research. The development of such tools is central to our mission as user facilities.”
Classifying local chemical environments
Different regions of the x-ray absorption spectrum are sensitive to different aspects of the material properties in a sample. For example, the x-ray absorption near-edge structure (XANES) focuses on the near-edge region of the spectrum, right above the onset energy sufficient to excite an electron from the inner shells of an atom to an empty state. XANES encodes rich information about the local chemical environment of absorbing atoms in a sample—including their geometric coordination, symmetry, and charge state (the number of electrons gained or lost from chemical bonding). But analyzing spectral data is very challenging because of their abstract nature.
“Unlike a microscope image of a material where you can directly see features like crystallinity or defects, XANES spectra encode information that requires domain expertise to interpret,” explained Lu.
Standard interpretation of signals in a XANES spectrum relies on characteristic features known as “fingerprints,” which are constructed from measurements on reference materials. However, this fingerprint approach fails when the sample is not a simple crystal and pertinent reference materials cannot be easily identified.
Large-scale theory-based simulations from atomic structure models can provide very useful insights for the interpretation of experimental XANES spectra; however, these simulations are often computationally expensive and time consuming, and their level of accuracy heavily depends on the chosen theoretical approximations and the system under study. As a result, robust spectral interpretation is currently the bottleneck of XAS studies. Furthermore, real-time interpretation of XAS spectra has emerged as a new challenge for studies of the dynamic evolution of materials under operating conditions and autonomous experimentation. The need for robust, efficient spectral interpretation is becoming increasingly widespread at synchrotron light sources.
“Real-time, accurate interpretation of x-ray scattering and spectroscopy measurements such as x-ray absorption, fluorescence, and diffraction is an important capability for users conducting research at NSLS-II and other synchrotron light facilities,” said Mehmet Topsakal, a scientific associate in the Materials for Energy Applications Group of Brookhaven’s Nuclear Science and Technology Department who is developing advanced data analysis and machine learning techniques for x-ray spectroscopy. “Every year, thousands of scientists from all over the world come to NSLS-II to probe the properties of various materials. A state-of-the-art spectral analysis pipeline would allow users to obtain useful feedback on their samples while experiments are ongoing and make adjustments on the fly to guide experiments. The question is, how can we do real-time spectral interpretation to uncover structure-spectrum correlations?”
Extracting information with machine learning
Leveraging big data and machine learning, Lu and Topsakal set out to answer this question with computational scientist Shinjae Yoo of Brookhaven Lab’s Computational Science Initiative (CSI) and Columbia University PhD candidate and DOE Computational Science Graduate Fellow Matthew Carbone.
“The DOE Computational Science Graduate Fellowship has afforded me a unique opportunity to extend beyond my chemical physics PhD research at Columbia to explore the power of machine learning algorithms, working alongside Brookhaven scientists,” said Carbone. “Machine learning leverages massive datasets to build highly perceptive models that, once trained, can make on-the-fly predictions on new data. Such models could be used to bypass expensive quantum chemistry calculations and support in operando material characterization.”
Members of this team and collaborators have been working on spectrum-to-structure and structure-to-spectrum mappings for several years. In 2017, they developed machine learning models to predict the average coordination numbers of metal nanoparticles from XANES spectra. Last year, they created a XANES database to resolve the local structure of an amorphous titanium-oxide coating for photocatalytic applications. They also built a machine learning model capable of predicting the local symmetry of absorber atoms from simulated XANES spectra of transition-metal oxides.
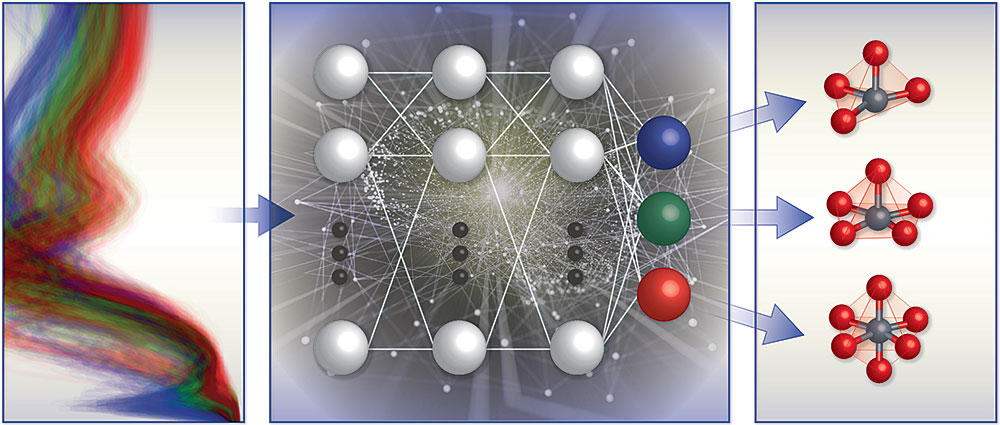
A schematic illustration of the team's spectrum-based local chemical environment classification framework. They trained machine learning models (middle) with computational x-ray absorption spectra database (left) to predict the local geometry around positively charged transition metal ions (right).
“When performing spectral interpretation based on domain expertise, we tend to focus on specific features engineered from our intuition,” said Lu. “Machine learning can extract the information we need in a statistically salient way that eliminates human bias.”
Predicting x-ray absorption spectra
Building on their past successes, the team took on a more challenging problem: train a machine learning model to quickly predict spectra based on known molecular structures. Such a model would bypass the need for computationally expensive simulations, which are not feasible during operando experiments, when scientists are studying materials under operating conditions. Despite growing machine learning efforts to predict the chemical properties of materials, direct predictions of the spectral functions of real materials had not yet been achieved.
“One technical difficulty is building an optimal representation of molecular structures that can code the inherent symmetry of the molecules as input features for the machine learning model,” said Yoo.
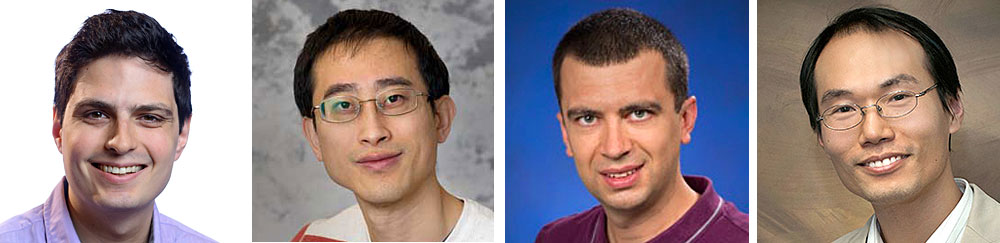
(Left to right) Matthew Carbone, Deyu Lu, Mehmet Topsakal, and Shinjae Yoo.
Adopting a recent idea proposed by scientists at Google, Topsakal and Carbone built a machine learning model based on a graph representation of molecules as the input, where atoms are represented as nodes and chemical bonds as edges.
“Computers can’t see molecules as we do,” said Topsakal. “A graph is a natural way to encode the structure and connectivity of a molecule—capturing which atoms are connected and the type and length of the chemical bonds connecting them. Moreover, this representation is invariant to transformations such as translations and rotations. This concept is analogous to that in image recognition, where an object such as a cat or dog in a background can still be classified correctly after the image is transformed.”
To train the model for a proof-of-principle demonstration, the team used a well-established database (called QM9) containing computed structural and chemical information on 134,000 small molecules with up to nine heavy atoms per atom type (carbon, nitrogen, oxygen, and fluorine). From this database, they selected two training subsets—one subset with molecules containing at least one oxygen atom, and another subset with molecules containing at least one nitrogen atom—and calculated their corresponding XANES spectra. Then, they used their trained models to predict the XANES spectra for oxygen and nitrogen absorption edges corresponding to excitations of electrons in the innermost shell of the respective atoms.
The machine learning model reproduced nearly all the significant absorption peaks and predicted the peak positions (energies at which peaks appear) and heights (absorption intensities) with very high accuracy. The model also automatically picked up on the domain knowledge that x-ray absorption spectroscopy is sensitive to functional groups, or groups of atoms with similar chemical properties and reactivity. Depending on which functional group the absorber atom belongs to, different features appear in the spectra.
“We’re the first to demonstrate that a machine learning model can be used to accurately predict full spectral functions of real physical systems directly from their structures,” said Topsakal. “Although we focused on x-ray absorption spectroscopy in our study, this method could be generalized to predict spectral information for other popular techniques, including infrared and gamma-ray spectroscopy.”
“Once we train the machine learning model, we do not need to run time-consuming physical simulations, which take minutes, hours, or even days,” said Yoo. “We enabled not only real-time spectra prediction but also the simultaneous generation of hundreds and thousands of spectra inferences by using multiple graphics processing units, or GPUs. Such technology is key to enabling automated beamline controls and accelerating scientific discovery. Combined with methods to sample material structures, such models can be used to quickly screen relevant structures to drive material design and discovery.”
Next, the team would like to combine concepts from their model that predicts local symmetry from XANES spectra and this new model that predicts XANES spectra from molecular structures. Ultimately, their goal is to extract more comprehensive information about the local chemical environment or even the structure of entire molecules from experimental measurements.
“Machine learning tools, such as those for image and speech recognition and drug discovery, are under rapid development,” said Lu. “The key is figuring out how to adapt these tools in an innovative way to tackle materials science problems.”
“Our goal in developing artificial intelligence and machine learning technologies is to solve unique scientific challenges by both adopting the latest technology breakthroughs in these areas and coming up with novel approaches that contribute back to the respective research communities,” added Yoo.
This work was funded by the DOE Office of Science and used resources of Brookhaven Lab’s Scientific Data and Computing Center, part of CSI. The DOE Computational Science Graduate Fellowship is supported by the DOE Office of Science and National Nuclear Security Administration.
Brookhaven National Laboratory is supported by the U.S. Department of Energy’s Office of Science. The Office of Science is the single largest supporter of basic research in the physical sciences in the United States and is working to address some of the most pressing challenges of our time. For more information, visit https://energy.gov/science.
Follow @BrookhavenLab on Twitter or find us on Facebook.
2020-17215 | INT/EXT | Newsroom