Argonne Scientists Help Explain Phenomenon in Hardware That Could Revolutionize AI
A team of scientists from national laboratories and universities has developed a device that can sort information similarly to the most sophisticated machine known to mankind: the human brain
March 15, 2021
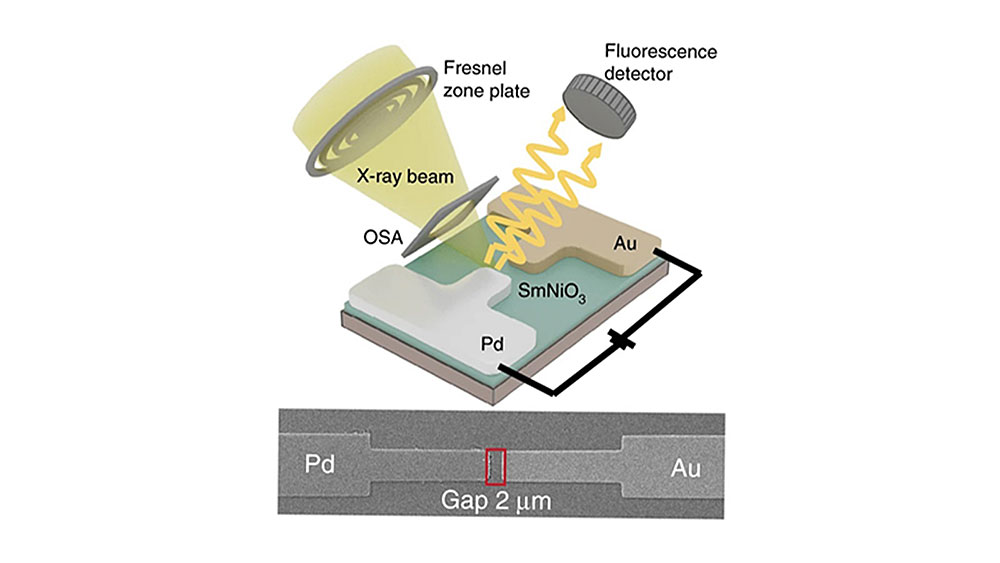
The collaborative research team utilized the powerful X-ray nanoprobe imaging tool to study the NdNiO3 device showing neuron tree-like memory. A scanning electron microscope image of the NdNiO3device is shown at the bottom. The red rectangle shows the scanned area of the X-ray imaging. (Image by Argonne National Laboratory.)
The following news release was originally issued by Argonne National Laboratory. The team of scientists from national laboratories and universities used the Hard X-ray Nanoprobe (HXN) beamline at the National Synchrotron Light Source II (NSLS-II). HXN offered the researchers the high spatial resolution and sensitivity needed to collect microscopic evidence for the origins of memory states in the hydrogen-doped nickelate memory devices. The HXN beamline is part of the imaging and microscopy program at NSLS-II, a U.S. Department of Energy (DOE) Office of Science User Facility located at DOE's Brookhaven National Laboratory. For more information about Brookhaven's role in this research, contact Cara Laasch, laasch@bnl.gov.
By Jo Napolitano, Argonne National Laboratory
Artificial intelligence, or AI, requires a huge amount of computing power, and versatile hardware to support that power. But most AI-supportive hardware is built around the same decades-old technology, and still a long way from emulating the neural activity in the human brain.
In an effort to solve this problem, a group of scientists from around the country, led by Prof. Shriram Ramanathan of Purdue University, has discovered a way to make the hardware more efficient and sustainable.
Ramanathan and his team used quantum materials — those whose properties operate outside the bounds of classical physics — to develop a device that can sort information quickly and efficiently. Scientists at the Department of Energy’s (DOE) Argonne National Laboratory, DOE’s Brookhaven Laboratory (BNL) and the University of California, San Diego, helped him learn exactly how it works.
Ramanathan and his team began their experiment by introducing a proton into a quantum material called neodymium nickel oxide (NdNiO3).
They soon discovered that applying an electric pulse to the material moved the proton. They further learned that each new position of the proton created a different resistance state, which generated an information storage site called a memory state. Multiple electric pulses created a branch made up of memory states, mimicking the "tree-like" memory process of the human brain.
“This discovery opens up new frontiers for AI that have been largely ignored because the ability to implement this kind of intelligence into electronic hardware has not existed,” Ramanathan said.
He and his team chose to work with NdNiO3 because it exhibits unique electronic and magnetic properties. One of its most intriguing behaviors is its metal-to-insulator transition (MIT), for which the properties change dramatically from enabling free-flowing energy (like metal) to blocking the current (like ceramic or plastic) by changing temperature.
This unique MIT behavior has tremendous potential in electronic devices for computing and memory. In the current research, Ramanathan demonstrated the MIT process in NdNiO3 by doping protons into the material rather than by changing the temperature.
He and his team are the first to do this. Prior to the discovery, this kind of neuron “tree-like” network had only been observed in hardware operated at temperatures far too low for practical applications, somewhere between dry ice and liquid nitrogen.
After Ramanathan’s team made the device, scientists at the Advanced Photon Source (APS) and Center for Nanoscale Materials (CNM) — both DOE Office of Science User Facilities at Argonne — investigated the structural and electronic evolution in the material used to build it. Characterizations of the material and its working mechanism were conducted at APS beamlines 26-ID and 33-ID-D.
High-performance computing and AI applications based on current electronics consume a good deal of energy. This new artificially intelligent hardware will take some of that energy load off of those AI applications.
“We’re creating a hardware that could provide smarter algorithms for brain-like computing,” said co-author and physicist Hua Zhou of Argonne’s X-ray Science Division, who worked on this experiment at the APS. “In fact, the energy demand will be cut significantly using this technology.”
Potential applications include those related to neuromorphic computing systems, those that can learn and perform tasks on their own by interacting with their surroundings, and artificial synapses, which emulate biological synaptic signals in neuromorphic systems to attain brain-like computation and autonomous learning behaviors. Neuromorphic memory systems and artificial synapses could help make more energy efficient and smarter AI chips, which are used in both consumer and industrial electronics.
Findings in this area could also improve biosensing, which is critical to medical diagnostics.
Researchers at the University of California, San Diego, characterized the device at the microscopic scale utilizing hard X-ray nanoprobe tools at both APS and the National Synchrotron Light Source II (NSLS-II), a DOE Office of Science User Facility at BNL.
The team used CNM’s high-performance computing cluster to investigate the atomistic mechanisms behind the tree-like behavior in nickelates.
“Using the high-performance computing cluster at CNM, we showed how the presence of an electric field can dramatically alter the barrier associated with proton migration in nickelates,” said Sukriti Manna, lead computational author and a postdoctoral researcher at the University of Illinois at Chicago (UIC) and Argonne. Manna performed the quantum calculations needed to unravel the mystery behind this phenomenon.
“An important aspect of the tree is to understand the atomistic mechanisms that enable branching,” said Subramanian Sankaranarayanan, associate professor at UIC and theory group leader at CNM. “In simple terms, each branch of the tree is likely a different proton migration pathway controlled by electric fields.”
Sankaranarayanan said the sharing of intelligence features between hardware and software will be particularly useful in advanced applications, such as those related to self-driving cars or in the discovery of life-saving drugs.
“We are incredibly proud of our role in unlocking the potential of this critical discovery,” he said.
The results of this study are published in the journal Nature Communications.
2021-18769 | INT/EXT | Newsroom