A Simulation Success Story
Computational modeling offers new views of Covid and a peek into the future of research
March 15, 2022
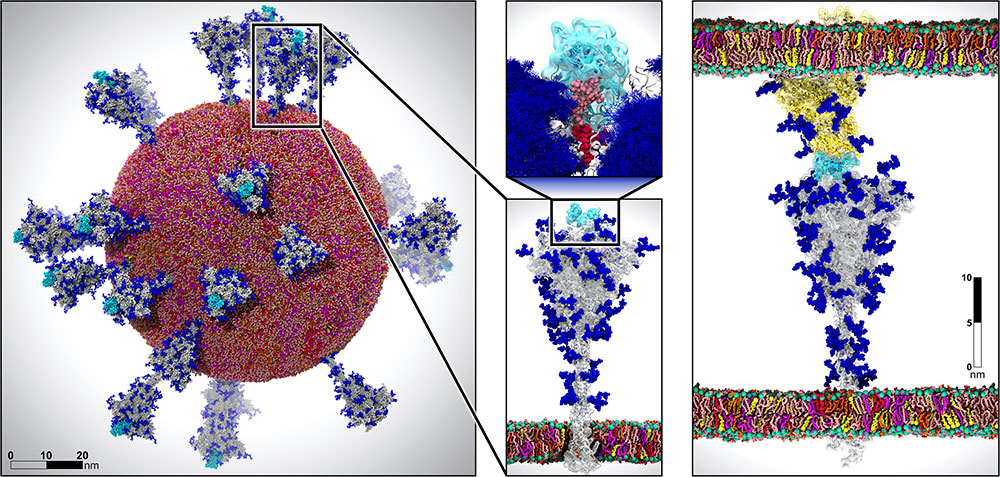
Multiscale SARS-CoV-2 model that features 305 million atoms (left), making it one of the largest biological systems ever simulated with such accuracy. Image from DOI: 10.1177/10943420211006452
As the effort to combat the SARS-CoV-2 virus began, among the most pressing questions were how to gauge its infectivity and get a better picture of those dynamics from the inside out. Answering them initially would bring together nearly 30 researchers, representing a dozen institutions and nearly as many disciplines, including scientists from the Computational Science Initiative (CSI) at the U.S. Department of Energy’s (DOE) Brookhaven National Laboratory.
Matteo Turilli and Shantenu Jha, from CSI’s Computation and Data Driven Discovery (C3D) department, would provide new computational improvements that incorporated artificial intelligence (AI)-based methods as part of an integrated workflow used to generate simulated views of SARS-CoV-2 that otherwise could never be achieved solely by experiment. Their work with the multidisciplinary collective has yielded awards, accolades and, more importantly, progress both in mitigating the damage wrought by a roughly 0.1 micron-sized particle and in changing how scientists approach discovery.
Year 1: On a Mission
While small in stature, SARS-CoV-2 decidedly has packed a big punch. This extended to its simulation. In 2020, the group, including Turilli and Jha, was seeking to generate simulations that would afford a more intricate understanding of the virus’ structure and dynamics to see how it moves, responds and infects a host. The work focused on modeling the spike protein, its main infection mechanism, using all-atom molecular dynamics (MD) simulations that can show biological systems at the atomic level and available (yet diverse) experimental data sets.
Because the team—a mix of computer scientists, biologists, physicists, chemists and mathematicians—was dealing with different models and vast data sets with no natural connectivity, even a powerful high-performance computing (HPC) system eventually would top out and start to experience various degrees of processing lag well before any useful resolution was achieved.
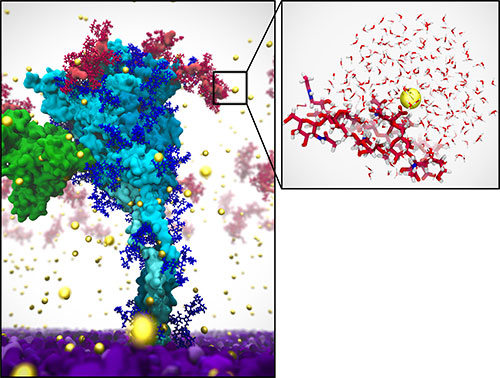
This image of the SARS-CoV-2 delta spike protein is a direct result of the multiscale, multi-surrogate research approach used to model the virus' dynamics. It required coupling classical and quantum computing enhanced and connected by artificial intelligence, which was enabled by computational improvements done at Brookhaven Lab. Image from #COVIDisAirborne.
“Our question was understanding and explaining how to build up these multiscale models that lacked any prescriptions for coupling,” explained Jha, who leads C3D at Brookhaven Lab CSI and is a professor of computer engineering at Rutgers University. “Each method was supplemented by advanced machine learning, and we helped build the software systems to run them at scale.”
Another problem for multiscale simulations is that they tend to be specified, for example, either only for MD, quantum, or coarse-grained models. One version may not understand the physics or provide accuracy at specific timescales. Thus, Turilli and Jha had to create the “computational glue” to replicate and simulate a “real” SARS-CoV-2 environment.
“The real challenge was posed by the different tasks from the computational point of view,” said Turilli, a scientist in CSI’s C3D group. “For some tasks, the problem was scale. For others, it was the heterogeneity of using different machines. Simply coordinating all of these things posed a grand challenge, one that goes beyond COVID-19 research. Advancing these pipelines, these workflow ecosystems, is a type of application with multiple impacts in multiple domains.”
To that end, they focused on improving the software that would integrate, port and scale the disparate models and data to provide the sophisticated workflow required to simulate the SARS-CoV-2’s spike protein dynamics in a useful, timely way. Their effort involved augmenting software developed at Brookhaven Lab, including DeepDriveMD, a framework that coordinates parallel execution of ensemble simulations and drives them with AI models. According to Jha, DeepDriveMD builds on traditional HPC simulations enhanced by machine learning. For this effort, DeepDriveMD was engaged in folding protein simulations, providing one order of magnitude sampling efficiency. More directly, it sped things along.
The complicated endeavor was well worth it as the team was able to complete a SARS-CoV-2 simulation that contained 305 million atoms, one of the largest biological systems ever simulated. Their simulations provided never-before-available, accurate views of the spike protein’s full glycan shield, revealing how glycans (carbohydrate-based polymers that play key biological roles) regulate its infectivity. The simulations also showed interactions between the spike and ACE2 receptor, the enzyme that provides the entryway for SARS-CoV-2 in the human body.
While insights from this substantial SARS-CoV-2 simulation could help add efficiency to drug discovery and therapeutics research, the AI-driven workflow itself was a step forward in informing how to couple large-scale calculations gleaned using classical, less costly computing at one institution with others completed via more computationally demanding quantum methods at another. It also posed novel challenges to the nation’s most powerful computing systems. Even using AI methods to integrate the models and data then drive the knowledge gained from one scale to another, the team could not have realized such simulations without the compute capabilities provided by Summit, an IBM-built system at DOE’s Oak Ridge National Laboratory (ORNL) and currently the second most powerful supercomputer in the world.
“While the bulk of the work was on Summit, there were a variety of machines, from university clusters to the NSF [National Science Foundation]’s Frontera at TACC [Texas Advanced Computing Center], that were used along the way for the various models.” Jha added. “Only DOE’s big machines could support the full degree of coupling of machine learning and HPC simulations.”
Notably, the team collected the details from their work on Summit, including how the optimized and scaled MD code exhibited strong scaling across all of the system’s GPUs (27,648 NVIDIA Volta V100s), which became an integral reason they were awarded the first-ever Gordon Bell COVID-19 Special Prize at SC20.
Year 2: A New Variant
By 2021, the award-winning collaboration was engaged on a new front, seeking an even closer look at the increased transmissive structure of the entire SARS-CoV-2 delta variant, namely affording atomic-level views of its construction and dynamics once the virus is inside respiratory aerosol particles.
“Remember in the early days of the pandemic, you were bringing in groceries and disinfecting them?” Jha said. “We did not know enough about its surface [life]. Now, we do. We also know Covid is airborne, and it allows it to become more potent. Hence, we doubled-down on masks and social distancing.”
“You need multidisciplinary teams to tackle these problems and scales and provide rapid, actionable results. Single-genius science is behind us. We need numerous people involved to tackle these problems that are too big to tackle alone.”
Shantenu Jha, Computation and Data Driven Discovery Chair at Brookhaven Lab and professor of computer engineering at Rutgers University
As with their earlier simulations, the team was seeking to answer the first-principles questions and validate experimental data. Their aerosol simulations would use physics-based approaches to investigate areas that could not be achieved by experiment and would amplify understanding of airborne viral transmission.
Unlike their initial efforts in 2020, getting the computational parts working together correctly proved more complicated. Again, they employed AI coupled to HPC at multiple levels, including coupling WESTPA (Weighted Ensemble Simulation Toolkit with Parallelization and Analysis) software to DeepDriveMD, which was executed on Summit and provided a 100 times improvement in the exploration of phase space. In addition, the team employed cloud computing, which proved useful in decreasing both time to solution and computational costs.
As the team recorded in its paper about the work, their success in building an AI-based multiscale framework to enable simulation of aerosol particle dynamics was tempered in that it also exposed “weaknesses in the HPC ecosystem,” which will pose challenges to building on and using such technologies in the long run. Still, the team’s work was acknowledged for its innovation by being named a finalist for the Gordon Bell COVID-19 Special Prize in 2021.
Not Over Yet
While COVID-19 continues to cast a long shadow over the world, one of the bright spots is how the scientific community rapidly came together, amassing techniques and tools that immediately were put to work—and often openly shared—to enhance scientific discovery.
DeepDriveMD is an example of software that can be adapted to solve similar problems as those posed by COVID-19, especially as its function and performance needs are upgraded. Jha noted that both ORNL and Indiana University are investigating DeepDriveMD’s applicability to materials research.
“Putting the software to use makes it better,” he said. “Implementation is improving it along with machine learning and simulation overall.”
Turilli praised the “heroic effort” it took to bring all of the disparate science domains and researchers together to take on these challenging causes, noting that this style of research should be supported by default.
“We should make these efforts consistent, large and routine—an everyday research tool to benefit society,” he said.
Jha could only agree: “The next paradigm of computer science is here. Covid is just one problem to work on, but can you build up the infrastructure for broad analysis? Will it allow you to do something or otherwise advance methods to new scales? The #COVIDisAirborne effort offered a sneak preview of the new computational science research. You’re not doing research if you aren’t doing it collectively.
“You need multidisciplinary teams to tackle these problems and scales and provide rapid, actionable results,” he concluded. “Single-genius science is behind us. We need numerous people involved to tackle these problems that are too big to tackle alone.”
Brookhaven National Laboratory is supported by the Office of Science of the U.S. Department of Energy. The Office of Science is the single largest supporter of basic research in the physical sciences in the United States and is working to address some of the most pressing challenges of our time. For more information, visit science.energy.gov.
Follow @BrookhavenLab on Twitter or find us on Facebook.
Related Links
- Casalino, Lorenzo, et al. “AI-Driven Multiscale Simulations Illuminate Mechanisms of SARS-CoV-2 Spike Dynamics.” The International Journal of High Performance Computing Applications, vol. 35, no. 5, Sept. 2021, pp. 432–451, doi: 10.1177/10943420211006452.
Winner: ACM Gordon Bell Special Prize for High Performance Computing-based COVID-19 Research, SC20 - Dommer, Abigail, et al. “#COVIDisAirborne: AI-Enabled Multiscale Computational Microscopy of Delta SARS-CoV-2 in a Respiratory Aerosol.” bioRxiv 2021.11.12.468428 preprint, Nov. 2021, doi: 10.1101/2021.11.12.468428.
Finalist: ACM Gordon Bell Special Prize for High Performance Computing-based COVID-19 Research, SC21 - Screening Study IDs Inhibitor of Key COVID Virus Enzyme
2022-19440 | INT/EXT | Newsroom