Self-Assembly Theory and COVID-19 Modeling with Alexei Tkachenko
interview with a CFN staff member
May 23, 2022
Soft condensed matter theorist Alexei Tkachenko, a member of the CFN Theory and Computation group, works at the intersection of physics and computation. His work combines statistical physics and computer simulations to explore self-assembly, the phenomenon that allows materials to self-build into highly organized, often very intriguing structures. Some of his recent work involves using DNA strands to facilitate self-assembly. He is also interested in statistical mechanics, condensed matter and biophysics, and recently has begun to apply his expertise to epidemic modeling, a shift motivated by the global COVID-19 pandemic. Tkachenko has been a CFN staff member since 2009. He has a Ph.D. in Physics from Bar-Ilan University (Israel) and a B.Sc/M.Sc in Theoretical Physics from the Moscow Institute of Science and Technology (Russia).
What kind of work do you focus on at CFN?
My main interest has been in the field of self-assembly—designing nanoscale building blocks that assemble into ordered structures by themselves. Self-assembled structures have the potential to lead to a wide array of new technologies, from tiny electronics to sensors.
I have studied a few approaches to this. One promising idea is the use of synthetic DNA strands to assist self-assembly. The DNA does not serve a biological function in this application, but rather is used to guide the building blocks. I am on the theoretical side of this; my job is to show the potential of an approach using computational methods and then work with the experimentalists to try to make it happen.
How can DNA assist in self-assembly?
What we’ve done is take gold nanoparticles and give them “hair” using single strands of DNA that are attached to the nanoparticles at certain locations. The idea is that the nanoparticles can be programmed to self-assemble based on the way that DNA interacts with other strands of DNA. Using this approach, scientists can get the nanoparticles to assemble into structures that would have been very difficult or impossible using other methods.
Tell us about your pandemic modeling research. What is your motivation for modeling COVID-19 trends?
With the pandemic already past the two-year mark—much longer than anyone thought it would last—scientists have become more invested in gaining a better understanding of the factors that govern the waves and plateaus of infection rates. We want to be able to better predict what future outbreaks of COVID-19 will look like, and even apply that knowledge to future epidemics. This means that epidemiological models need to be improved: They need to be much more complex to better reflect the complexity of human interaction. With this work, I hope to help make these models better.
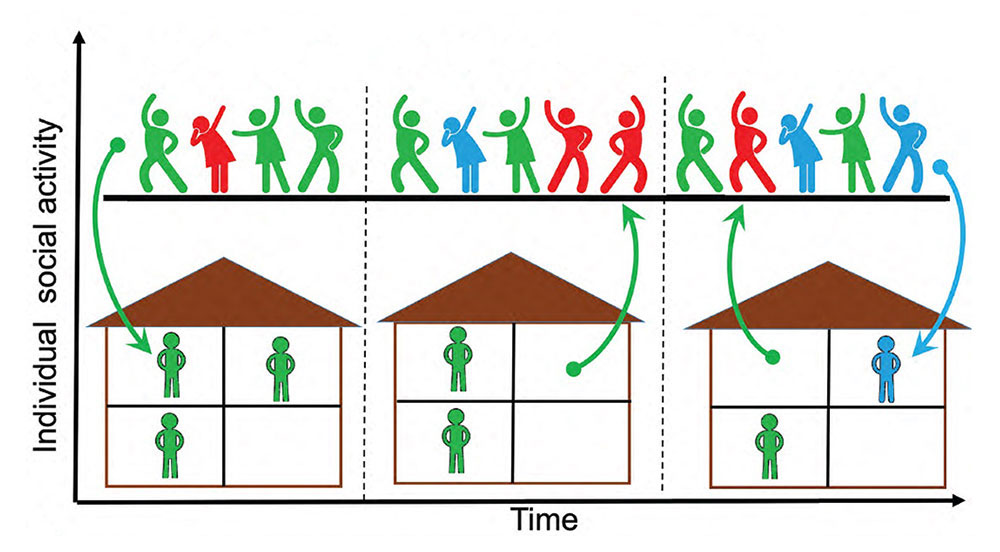
Schematic illustration of the Stochastic Social Activity model in which each individual changes their social activity over time. People with low social activity (depicted as socially isolated figures at home) occasionally increase their level of activity (depicted as at a party). The average activity in the population remains the same over time, but individuals constantly change their activity levels from low to high (arrows pointing up) and back (arrows pointing down). Individuals are colored according to their state: susceptible = green, infected = red, and recovered/removed = blue. The epidemic is fueled by constant replenishment of the susceptible population when individuals move from the low-activity state to high-activity settings.
How does your latest work address the pandemic?
I worked on a team of scientists that developed a model that encompasses the randomness and always-changing social interactions between individuals, as well as takes into account the differences in the size of social networks. We based our model on data taken from four U.S. regions prior to the rollout of vaccines. We found that adding a factor that accounts for randomness in human social behavior will always produce waves or plateaus of infections—and that this occurs whether or not the model also accounts for how people change their social behavior based on knowledge of current infection rates. This is a continuation of modeling work that we published in April of 2021, which essentially showed that a temporary state of immunity emerged early on in the pandemic but dissolved—and led to future waves of infection—as people changed their social behaviors as the pandemic stretched out.
The expanded model also tells us, unfortunately, that COVID-19 may be here to stay. It indicates that it may become endemic in the global population, much like the common cold or the flu.
Can you elaborate more on why your model solves some issues in predicting the spread of infection?
The model describes three critical phenomena: why, during a pandemic, a wave stops; how it can progress at a nearly constant rate, forming a plateau; and why new pathogens actually stay with us permanently, entering what’s called an endemic state. This is an old mystery in epidemiology. Classical theory tells us when a new pathogen is introduced, it will eventually kill itself off by infecting enough people that herd immunity is developed, unless biological immunity is very short-lived. But even in the case where long-term biological immunity is developed, we explain the scenario of how a new pathogen stays endemic in a population.
What else did you discover that helps explain the establishment of an endemic?
We came up with a way to formulate the dynamics of an individual’s social networks and how that individual’s social activity fluctuates as a function of time. We account for the size of a person’s social networks and changes in activity over different time scales. Our approach yields a single number that captures these dynamics, and this number basically tells you how much the heterogeneity of social activity contributes to reducing the scale of the epidemic—or how soon a peak will be reached.
Brookhaven National Laboratory is supported by the U.S. Department of Energy’s Office of Science. The Office of Science is the single largest supporter of basic research in the physical sciences in the United States and is working to address some of the most pressing challenges of our time. For more information, visit https://energy.gov/science.
Follow @BrookhavenLab on Twitter or find us on Facebook.
2022-20612 | INT/EXT | Newsroom