Advanced Computing Lab Expands its Mission
Growing compute capabilities expand new collaborations within Brookhaven Lab and beyond
October 6, 2020
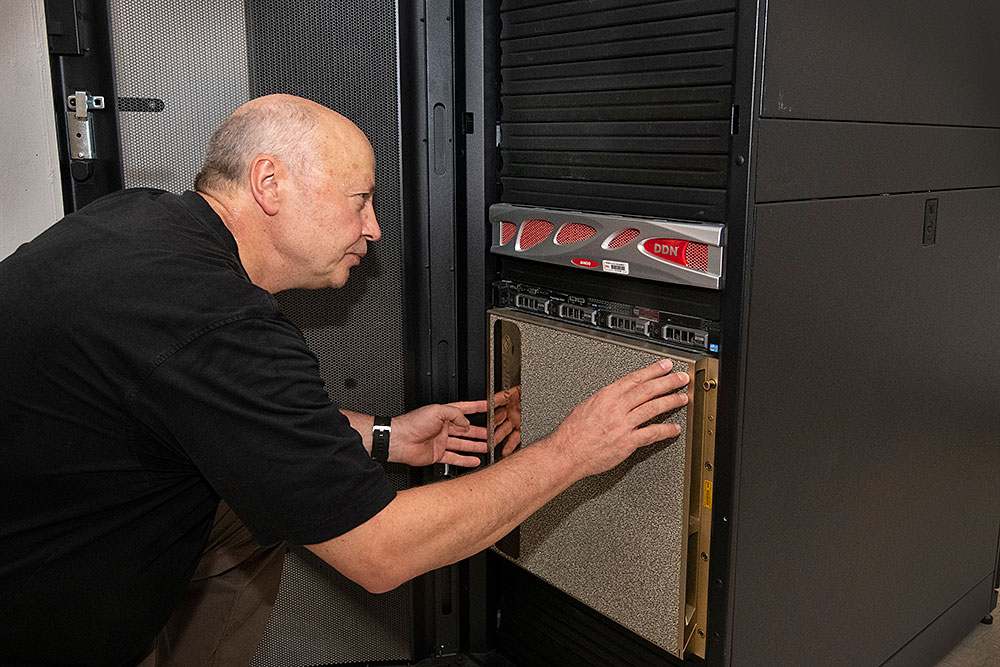
Adolfy Hoisie, Chair of CSI's Computing for National Security Department, oversees the Advanced Computing Lab. The ACL is part of CSI's overall renovation that aims to consolidate computing resources at Brookhaven Lab while also expanding hardware and software solutions for research, including enhancing big data analysis for scientific experiments.
As the Computational Science Initiative (CSI) at the U.S. Department of Energy’s (DOE) Brookhaven National Laboratory continues to expand its project portfolio and laboratory footprint, the Advanced Computing Lab, headed by Adolfy Hoisie, Chair of CSI’s Computing for National Security Department, is embarking on new endeavors aimed at building unique hardware and software solutions for fast analysis of data gleaned from scientific experiments.
CSI’s Advanced Computing Lab, called “ACL,” is designed as a focal point for research, development, testing, and testbed deployment of high-performance systems and software architectures. The goal is to focus on computing solutions for large-scale data-intensive workflows, machine learning, and artificial intelligence, or “AI.” These workflows, which monitor the setup and performance of a sequence of tasks, will be geared toward direct applications to science and national security challenges. CSI’s ACL provides a true collaborative environment, involving scientists and technologists from government laboratories, academia, and industry.
Centralizing Advanced Data Analysis
With on-campus scientific user resources such as National Synchrotron Light Source II (NSLS-II) and the Center for Functional Nanomaterials (CFN), both DOE Office of Science User Facilities, as well as the cryo-electron microscopy (cryo-EM) facility housed within the Laboratory for BioMolecular Structure, Brookhaven Lab is a hub for generating and processing experimental data. While many tools and methods have been introduced to address the challenges stemming from these complex, diverse, and extremely large datasets, the path from data to knowledge and understanding can be complicated and time-consuming.
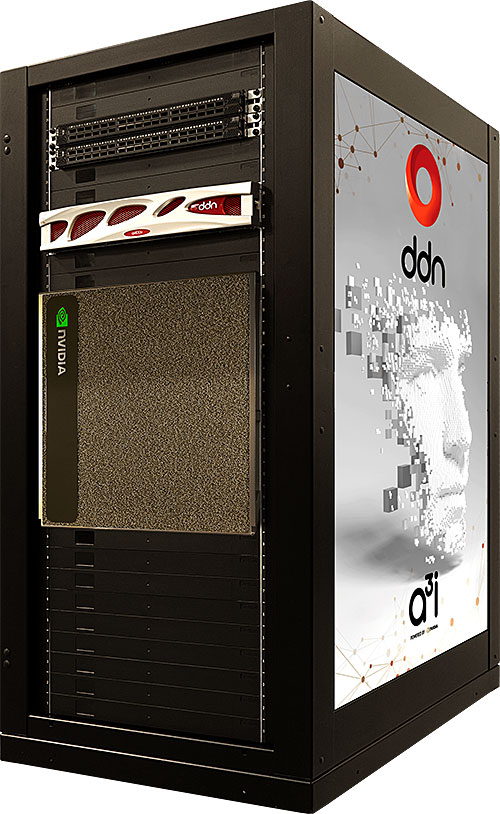
DDN's AI400X works in tandem with the NVIDIA DGX-2. This high-tech hardware is helping to expand the CSI Advanced Computing Lab's data analysis capabilities. (Photo courtesy of DDN)
To help manage this big data dilemma, CSI has adopted a strategic, Lab-wide approach to data analysis and management. In the near future, data originating from experimental facilities will be able to be transferred directly or from intermediate local storage to the Scientific Data and Computing Center (SDCC) through high-bandwidth dedicated networks. In the meantime, prototypes of production-grade SDCC systems are being deployed in the ACL.
“To provide analysis requirements in close to real time stretches the capabilities of current technologies,” Hoisie explained. “In a nutshell, we need to enable fast, accurate analysis and control of an experiment under very stringent performance goals, basically in a time frame that allows for analysis and action preferably while the experiment is still ongoing.”
The hardware architecture considered for such ACL projects features NVIDIA DGX-2 and DGX-A100 deep learning systems integrated with the AI400X, a brand new parallel file storage appliance from DDN designed for efficient performance and interoperability to accelerate even the most intensive system workloads that otherwise would strain a conventional system and impede progress.
“With its data paths and storage, DDN’s AI system allows for specialized, direct transfer from each of the GPUs [graphics processing units] in the NVIDIA box to storage,” Hoisie explained. “This new technology provides the ability for the DGX-2 to send data back and forth between storage and the GPUs, where the computational action happens. Having this technology as part of the ACL is a significant step forward toward the ability to analyze data from advanced cameras, trigger feedback loops for instrument re-setting, and provide overall optimal control of the experiment in close to real time.”
According to DDN, the AI400X was created for easy deployment, simplifying ongoing management and enabling tight integration into AI and analytics environments, like Brookhaven’s ACL, which are increasingly dominated by GPU-based compute systems. By creating a pre-configured standard appliance with its AI400X, DDN has removed the need for extensive design, planning, and tuning steps often associated with deploying a high-performance parallel file system, turning what previously may have been weeks of configuration into just a few hours of work.
“Our deep technical work with other industry leaders, like NVIDIA, and national laboratories, such as Brookhaven, means we are continuously exposed to complex challenges along the data path—not just to the edge of the storage system, but all the way to the application to ensure the most impact,” said Sven Oehme, Chief Research Officer at DDN. “With true end-to-end parallelism, complementary to the GPU architecture, the AI400X works in tandem with the NVIDIA DGX-2 to overcome traditional system bottlenecks. This technology paring accelerates even the most challenging data-intensive workloads, especially those associated with AI and deep learning, to generate fast and reliable results.”
New Hardware + New AI = Novel Analysis Opportunities
Expanding the ACL’s experimental facilities is part of CSI’s overall plan to better serve the needs of the scientific community both within and beyond Brookhaven Lab. Among its many research areas, CSI has emphasized optimal experimental design as a leading concern. Several projects are in progress that aim to improve techniques and computational capabilities to enhance how experiments are run for more precise outcomes, especially in areas that afford fast experimental setup—potentially even in operando.
“As we have expanded and diversified our research portfolio within CSI, we have realized that centralized experimental data analysis would have extensive benefits to the scientific community, especially as it aligns with our set goals for optimal experimental design,” said CSI Director Kerstin Kleese van Dam. “We will continue to pursue these areas as we evolve both our infrastructure and hardware capabilities.”
“This true-tech collaboration between Brookhaven Lab and industry partners like DDN shows how CSI is embracing an integrated approach to data analysis and discovery.”
Adolfy Hoisie, Chair of CSI’s Computing for National Security Department
According to Hoisie, experiments from CFN’s Electron Microscopy Facility may provide some of the first “real-life” test cases for the ACL. To meet CFN and other facilities growing data analysis challenges, Brookhaven’s data analysis ecosystem is being augmented with upgraded fiber bandwidth and switching infrastructure for fast data transfer from the instrument to the analysis system and storage.
Such high-volume and velocity data will be incorporated into a co-designed workflow, where system architecture. and analysis and control software are developed in tandem by CSI computer and data scientists and their research partners. As in numerous fields, novel machine learning and AI are the engine that will fuel the flexibility and application of the co-designed workflows.
More importantly as it relates to near-real-time experimental data analysis, AI can assist with deploying the necessary feedback loops tasked with reconfiguring an instrument while in operation by responding to specific experimental triggers. This ability would provide multiple benefits. For example, an experiment could be designed around real-time feedback to change experimental conditions on the fly, which would enable continuous data analysis and model generation as data are acquired. This “virtuous circle” from the instrument to the analysis and storage system and back to the instrument for timely reconfiguration would provide a significant productivity boost to experimental scientists.
According to Hoisie, the use cases provided through such collaborations would inform future production computing facilities in co-designing hardware and software systems for efficient and cost-effective analysis by addressing challenges that include processing statistically meaningful quantities of data, identifying key events in the data stream, and creating rapid data reduction protocols to drive real-time feedback to experimental conditions.
“This true-tech collaboration between Brookhaven Lab and industry partners like DDN shows how CSI is embracing an integrated approach to data analysis and discovery using leading-edge hardware and advanced methods in AI and machine learning,” Hoisie said. “The path to maximum scientific insight is no longer linear. Instead, we are working to design systems and workflows that add flexibility to scientific experiments and will aptly serve the real-life needs of research communities.”
Brookhaven National Laboratory is supported by the Office of Science of the U.S. Department of Energy. The Office of Science is the single largest supporter of basic research in the physical sciences in the United States and is working to address some of the most pressing challenges of our time. For more information, please visit science.energy.gov.
Follow @BrookhavenLab on Twitter or find us on Facebook.
2020-17350 | INT/EXT | Newsroom