Materials Discovery Through AI-powered Consensus
NSLS-II scientists developed an artificial intelligence (AI) ensemble for faster, more accurate data analysis
June 30, 2021
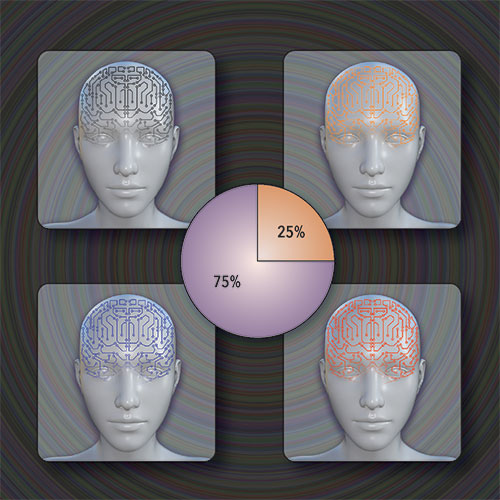
This image illustrates the result of the voting process performed by the different AIs within the ensemble. The researcher can use these results to inform further analysis of their x-ray diffraction data to answer scientific questions. Image credit: Brookhaven National Laboratory
The Science
Scientists developed an artificial intelligence (AI) ensemble for the autonomous characterization of x-ray diffraction (XRD) data and demonstrated its performance on organic and inorganic materials.
The Impact
While automation has accelerated the rate of measurement for materials discovery, the analysis techniques are still time-consuming and error-prone. This study offers researchers a fast classification tool to improve the accuracy of data analysis while saving time.
Summary
To develop new materials, such as better batteries, more cost-effective catalysts, or new pharmaceuticals, scientists first need to understand their inner makeup. One way to understand the nature of materials is phase identification using x-ray diffraction (XRD). Driven by the desire to accelerate materials discovery, researchers have developed highly automated data collection systems for XRD; however, this advancement has greatly outpaced XRD analysis techniques, which remain, for the most part, manual, time-consuming, error-prone, and impossible to scale.
In this study, a team of researchers have developed a new computer program, driven by artificial intelligence (AI), that can assist scientists in the classification of XRD patterns during the measurement. Their AI approach for the autonomous phase identification of diffraction patterns is accurate across both organic and inorganic materials, while the uncertainty of the classification is also known.
This novel AI approach uses an ensemble of AI agents, each of which classifies a material based on available data. Once each AI has voted, the researchers receive a report with potential material structures and their uncertainty, outlining the consensus the AIs have reached. This approach overcomes the overconfidence of traditional neural networks.
The team of researchers used the Pair Distribution Function (PDF) beamline to test the AI ensemble on a diverse set of organic and inorganic materials. They found that the AI ensemble is able to classify the materials and phases effectively, while allowing enough rooms for scrutiny by a human expert. The PDF beamline is part of a suite of advanced research tools for materials discovery at the National Synchrotron Light Source II (NSLS-II). NSLS-II is a U.S. Department of Energy (DOE) Office of Science user facility located at DOE’s Brookhaven National Laboratory, supporting a wide variety of researcher fields.
The developed program of this study will be part of the PDF beamline capabilities for materials discovery. In addition, the methodology of this study could be extended to any 1D response function in high-throughput materials research that requires classification and can be simulated at low cost and, therefore, could be expanded to several other available research tools at NSLS-II.
Download the research summary slide
Related Links
Press release: AI Agent Helps Identify Material Properties Faster
Related Brookhaven Press Release: Game on: Science Edition
Contact
Philip M. Maffettone
National Synchrotron Light Source II
pmaffetto@bnl.gov
Andrew I. Cooper
University of Liverpool, UK
aicooper@liverpool.ac.uk
Publications
P.M. Maffettone, L. Banko, P. Cui, Y. Lysogorskiy, M. A. Little, D. Olds, A. Ludwig, A. I. Cooper. Crystallography companion agent for high-throughput materials discovery. Nat. Comput. Sci. 1, 290–297 (2021). https://doi.org/10.1038/s43588-021-00059-2
Funding
We acknowledge financial support from the Engineering and Physical Sciences Research Council (EPSRC) (grant no. EP/N004884/1; P.M.M., M.A.L. and A.I.C.), BNL Laboratory Directed Research and Development (LDRD) projects 20-032 ‘Accelerating materials discovery with total scattering via machine learning’ (P.M.M. and D.O.), the Leverhulme Trust via the Leverhulme Research Centre for Functional Materials Design (P.C. and A.I.C.) and the German Research Foundation (DFG) as part of the Collaborative Research Centre TRR87/3 ‘Pulsed high power plasmas for the synthesis of nanostructured functional layers’ (SFB-TR 87), project C2 (L.B., Y.L. and A.L.). This research utilized the PDF (28-ID-1) Beamline and resources of the National Synchrotron Light Source II, a US Department of Energy (DOE) Office of Science User Facility operated for the DOE Office of Science by Brookhaven National Laboratory under contract no. DE-SC0012704. We thank ZGH (Zentrum für Grenzflächendominierte Höchstleistungswerkstoffe, Ruhr-Universität Bochum) and Diamond Light Source for access to beamlines I19 (MT15777) and I11 (EE17193) for XRD measurements.
2021-19013 | INT/EXT | Newsroom