An "Internet of Things" Approach to Enterprise Beamlines
Scientists propose a way to link AI-based instrument end stations designed for high-throughput studies of materials
June 14, 2023
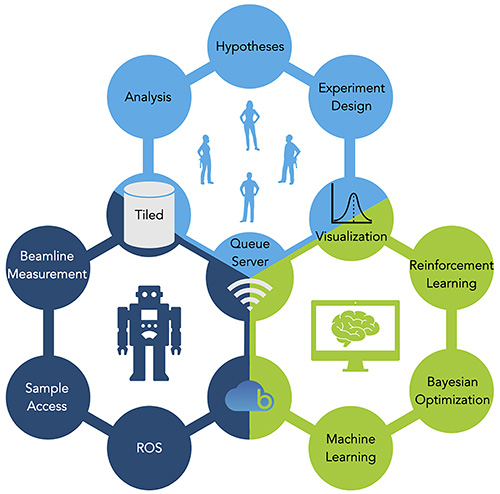
Schematic of the human-AI facility interface. Image credit: P. Maffettone, S. Campbell, M.D. Hanwell, S. Wilkins, D. Olds. Cell Reports Physical Science, 3 (11), 101112 (2022)
The Science
Scientists propose the world's first "internet of things" analysis engine to link multi-modal, self-driving (AI-based), enterprise beamlines, which are instrument end stations designed for high-throughput characterization and resolution of materials.
The Impact
Enterprise beamlines are a basis of next-generation materials acceleration platforms (MAPs), which are dedicated to the discovery of new materials. This proposal suggests an advanced MAP for more collaborative, efficient, impactful discovery.
Summary
Research hubs like the National Synchrotron Light Source II (NSLS-II), a U.S. Department of Energy Office of Science User Facility located at Brookhaven National Laboratory, are excellent locations for next-generation materials acceleration platforms (MAPs). MAPs, which are also known as self-driving laboratories, are designed to fast-track the discovery of new, useful materials. They are based on a few key principles, which include increased experimental throughput, structured data repositories, and establishing the best understanding of materials in order to efficiently optimize their properties.
At NSLS-II, future MAPs will be based on enterprise beamlines, which are instrument end stations that can automate routine materials characterization with high throughput and resolution. One key issue with current state-of-the-art MAPs, however, is that they tend to operate within isolated (and often outdated) infrastructure and do not support multi-modality.
In this study, researchers suggest an approach to changing this, one that takes advantage of what NSLS-II has to offer: extremely stable, bright photon beams; the data infrastructure to handle multi-scale, multi-modal, high-resolution studies on a variety of materials; and an experimental capacity that spans high-throughput routine measurements to specialized measurements at a best-in-class or only-in-class level.
The group suggests an “internet of things” for automated enterprise beamlines. It would merge core information technologies, robotics, and multi-modal artificial intelligence (AI). The goal is to maximize the full utility of light sources, collaborate with other MAPs, and, ultimately, yield discoveries that help secure the world’s energy future.
This “internet of things” analysis engine involves several components. It would orchestrate experiments across multiple end stations using the Bluesky data collection framework, a library for experiment control and collection of scientific data and metadata. The engine would also include flexible automation for sample manipulation and transport, programmed in the open-source Robotic Operating System, and would incorporate Bayesian optimization, a machine learning approach that enables efficient exploration of samples in real time and on multiple beamlines. The system would also include features, such as reinforcement learning, to optimize operations and make the best use of resources; additionally, it would include an interface to keep the human operators fully looped in.
This approach will leverage much of the existing hardware at NSLS-II for data collection. It could be deployed across all existing NSLS-II beamlines, with no need to construct new ones. Furthermore, all future beamlines would be able to adopt the technology.
Download the research summary slide (PDF)
Contact
Phillip Maffettone
Brookhaven National Laboratory
pmaffetto@bnl.gov
Publication
P. M. Maffettone, S. Campbell, M. D. Hanwell, S. Wilkins, D. Olds. Delivering real-time multi-modal materials analysis with enterprise beamlines. Cell Reports Physical Science 3(11), 101112 (2022). doi: 10.1016/j.xcrp.2022.101112
Funding
D.C., T.J., and A.S. contributed equally to this work. H.Z. acknowledge the financial support from National Science Foundation under Award Number CBET-ES-1924534. This research used 8-BM of the National Synchrotron Light Source II, a U.S. Department of Energy (DOE) Office of Science User Facility operated for the DOE Office of Science by Brookhaven National Laboratory under Contract No. DE-SC0012704. This research used resources 18-ID (FXI) of the National Synchrotron Light Source II, a U.S. Department of Energy (DOE) Office of Science User Facility operated for the DOE Office of Science by Brookhaven National Laboratory under Contract No. DE-SC0012704. The authors acknowledge the Boston Electron Microscopy Center at Northeastern University for the use of the SEM facility. The authors would like to acknowledge the Northeastern University Center for Renewable Energy Technology for the use of the XRD facility.
2023-21609 | INT/EXT | Newsroom