Smarter Experiments for Faster Materials Discovery
Scientists created a new AI algorithm for making measurement decisions; autonomous approach could revolutionize scientific experiments
September 30, 2019
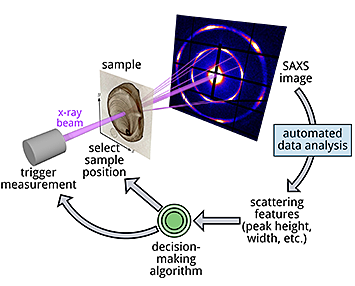
Scientists developed an algorithm that receives real-time analyzed data from the last measurement step, adds this data to its model, calculates the best next step, and sends its decision to the beamline to execute the next measurement.
The Science
Scientists created and tested the Surrogate Model Autonomous expeRimenT (SMART), a new algorithm and form of artificial intelligence (AI), that can make autonomous decisions to define and perform the next step of an experiment without human interaction at an x-ray scattering instrument.
The Impact
By improving the way researchers do their experiments, they are liberated from micro-managing their experiments and can tackle more complex challenges in a faster and more efficient way, leading to quicker materials discovery for new technologies.
Summary
Modern scientific experiments are increasingly complex, requiring scientists to explore innumerable different conditions in their pursuit of next-generation materials. Scientists at Brookhaven National Laboratory and Lawrence Berkeley National Laboratory have developed new AI methods to enable “autonomous experimentation.” In autonomous experiments, scientific instruments can run without human intervention, automatically collecting data and deciding what measurement to collect next. This liberates the human scientist to focus on high-level scientific understanding.
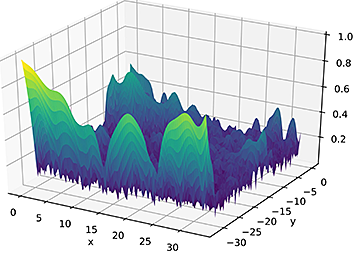
Typical error functions, where high regions indicate goods spots to perform a next measurement. (Credit: Marcus Noack, Berkeley Lab)
This collaboration demonstrated the effectiveness of SMART, the new algorithm, for autonomous decision making. The algorithm selects what data to collect by calculating uncertainty and the expected knowledge gain for possible future measurements. In other words, it estimates what follow-up experiment will teach researchers the most about a scientific problem at hand and then automatically launches that experiment. This AI algorithm runs iteratively, collecting data in a highly efficient manner and thereby automatically reconstructing a useful map of the parameter space being explored.
In addition to developing this general and flexible algorithm, the team deployed the method at an x-ray scattering beamline at Brookhaven National Laboratory. The Complex Materials Scattering (CMS) beamline is operated in partnership between the National Synchrotron Light Source II (NSLS-II) and the Center for Functional Nanomaterials (CFN). The CMS beamline was used to implement this methodology, where the autonomous algorithm selected the sequence of measurements to perform on real material science samples.
Download the research summary slide
Related Links
- Brookhaven Lab Feature Story: “Smarter Experiments for Faster Materials Discovery”
- Berkeley Lab Feature Story: “SMART Algorithm Makes Beamline Data Collection Smarter”
Contact
Marcus Noack
Lawrence Berkeley National Laboratory
MarcusNoack@lbl.gov
Kevin Yager
Brookhaven National Laboratory
kyager@bnl.gov
Masafumi Fukuto
Brookhaven National Laboratory
fukuto@bnl.gov
Publications
M. M. Noack, K. G. Yager, M. Fukuto, G. S. Doerk, R. Li, J. A. Sethian, “A Kriging-Based Approach to Autonomous Experimentation with Applications to X-Ray Scattering”, Scientific Reports 9, 11809 (2019). DOI: 10.1038/s41598-019-48114-3
Funding
The work was partially funded through the Center for Advanced Mathematics for Energy Research Applications (CAMERA), which is jointly funded by the Advanced Scientific Computing Research (ASCR) and Basic Energy Sciences (BES) within the Department of Energy’s Office of Science, under Contract No. DE-AC02-05CH11231. This work was conducted at Lawrence Berkeley National Laboratory and Brookhaven National Laboratory. This research used resources of the Center for Functional Nanomaterials and the National Synchrotron Light Source II, which are U.S. DOE Office of Science Facilities, at Brookhaven National Laboratory under Contract No. DE-SC0012704.
2019-16848 | INT/EXT | Newsroom