
Climate and Process Modeling
Research on Renewables
There have been growing demands for various forms of renewable energy, including solar energy and wind energy, both nationally and internationally. For example, US. DOE has heavily invested in the related areas through the EERE Solar Energy Technology Office and Wind Energy Technologies Office. The New Vision of New York State for renewal energy demands that 50% of electricity must come from renewable sources.
Renewal energy generation is different from many traditional energy sources in that its availability depends on highly varying atmospheric phenomena such as solar irradiance, wind, clouds, and precipitation. For example, a small improvement (around 10%–20% improvement in mean absolute error) in forecasting winds can lead to millions of dollars in cost savings. Since total electric supply and demand must always remain in sync, the cost-efficient integration of such renewables in the electric grid ultimately depends on our ability to accurately forecast related key meteorological variables − solar irradiance for solar farms and wind for wind farms − over various time horizons (e.g., mins, hours, and days ahead), and at high space-time resolutions. Furthermore, the so-called ramp (extreme event) forecast is critical for improving renewal energy forecasts.
Such needs pose new challenges, perhaps greater than conventional weather and climate forecasting, in areas of both atmospheric (physical) and computational sciences. For example, (1) the necessity of forecasting extreme events, at high-resolutions, and over multiple time horizons calls for better representation of unresolved subgrid processes, especially cloud microphysics, radiative transfer, planetary boundary layer, turbulence, and atmosphere-surface interactions. (2) Development of accurate physical models also requires high-resolution measurements and their assimilation into physical models. (3) Both high resolution measurements and high-resolution physical modeling generate an explosive growth of data characterized by large volume, variety, velocity, and veracity. The so-called big data science is causing revolutionary changes in many areas including science and technology. Data-driven modeling based on advanced machine learning (ML) has emerged as a hot topic in various fields. Limited studies have demonstrated that recent advances in data-driven models can be effectively used to improve short-term nowcasting, develop ML emulator for physical models or process parameterizations, and enhance/accelerate data assimilation. The Climate and Process Modeling group, in collaboration with scientists at National Renewable Energy Laboratory), SUNY at Albany), SUNY at Stony Brook), and BNL Computational Science Initiative), are addressing these challenges by capitalizing on the great advances in physical models, machine learning, and measurements through two projects briefly described here.
The multi-institution project “Advancing the WRF-Solar Model to Improve Solar Irradiance Forecast in Cloudy Environments”, supported by the EERE Solar Energy Technology Office (SETO), aims to improve the state-of-the-art WRF-Solar model for solar irradiance forecasting, including Global Horizontal Irradiance (GHI), Direct Normal Irradiance (DNI), and thus Diffuse Horizontal Irradiance (DHI), in cloudy conditions. The project has four objectives: 1) improving cloud microphysics parameterizations; 2) improving radiative transfer; 3) developing an innovative analysis package; 4) performing model evaluation against measurements to ascertain the model improvement. Five efforts will be conducted to achieve these objectives and goal, which are illustrated in Figure 1.
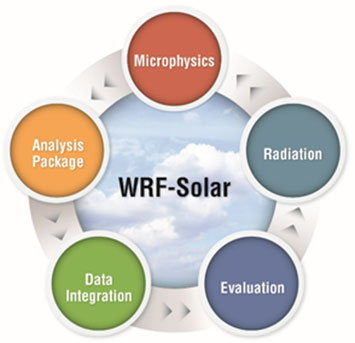
Figure 1. Illustration of the five interconnected tasks. Model improvement involves iterative cycle of parameterization/model development, evaluation against measurements to identify model deficiencies and further improvement. The microphysics task focuses on implementing/testing and improving the unique microphysics parameterizations developed at BNL; the radiation task focuses on improving the partitioning of the total solar irradiance into direct and diffuse irradiances; a WRF-Solar suite consisting of the nested WRF, its large eddy simulation version (WRF-Solar-LES), and single column version (SWRF-Solar) is used in model evaluation, along with innovative analysis package based on cloud-radiation relationships and big data sciences (machine learning and online streaming).
The other project “Building an Integrative Forecast System to Address Challenges Facing Renewable Energy Forecast” is a cross-directory LDRD project between CESD and CSI, aiming at developing a novel integrative system for forecasting renewable energy by seamlessly integrating a numerical weather prediction model (WRF-Solar), machine learning models, and measurements. The primary target is at forecasting wind for offshore and land-based wind farms. The project has four primary objectives: 1) Develop a physics-based forecast system by improving the representation of the physical processes essential to improving wind forecasting, including planetary boundary layer (PBL), turbulence, shallow convection, and land surface processes; 2) Develop a data-driven forecasting system; 3) Integrate the physics-based and data-driven models as an integrative forecasting system. 4) Prepare state of art high-resolution 4D data and perform model evaluation to quantify the model accuracy and uncertainty.
Through the two projects, we expect to improve the WRF parameterizations for several key physical processes essential to improving forecasting solar and wind energies, including cloud microphysics, radiative transfer, PBL, and turbulence processes. Furthermore, since the physics-based and data-driven forecast models likely have their sweet spots of forecasting lead time (e.g., data-driven machine learning models are likely better for short-term nowcasting), optimal blending of the two types of models in the integrative forecast system are expected to improve wind forecasting over a range of horizons. Because data-physics “hybrid” modeling is largely uncharted terrain, seamless integration of ML models with WRF models presents a scientifically interesting and exciting opportunity for novel and important contributions to forecasting. There are many topics involved here that will be the subject of research: the details of data-assimilation in this hybrid scheme, how to build a high-resolution emulator that acts as a surrogate for high-resolution WRF, and how to efficiently perform uncertainty quantification with such a hybrid model. It should be emphasized that what we are proposing here -- seamless integration of data-driven and physics-based approaches to forecasting -- could represent a significant breakthrough in the drive to develop “physics-aware” machine learning models.
Adequate model evaluation to quantify forecast accuracy, data assimilation, and training/testing ML models all call for high-resolution, high quality measurements. Both projects will analyze and integrate the New York State Mesonet measurements (http://nysmesonet.org/), along with other existing data sets, to generate a 4D dataset tailored for specific purposes, and in context of utilizing the WRF-Solar suite and big data sciences illustrated in Figure 2.
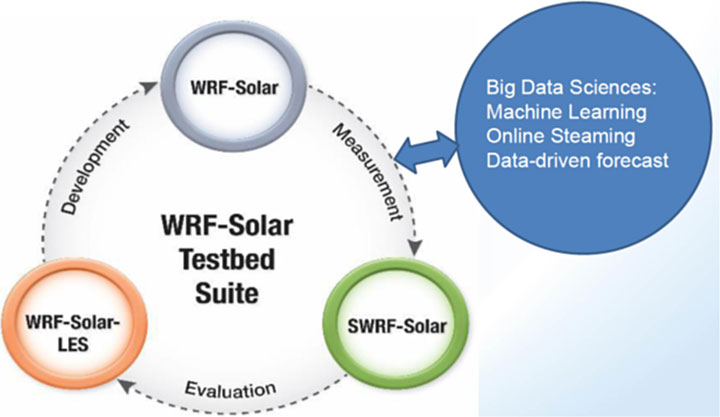
Figure 2. Illustration of the WRF-Solar suite, and its integration with the big data sciences including machine learning, online streaming and data-driven forecast.