Scientific Highlights
July 12, 2022
Datacentric Machine Learning in Quantum Information Science
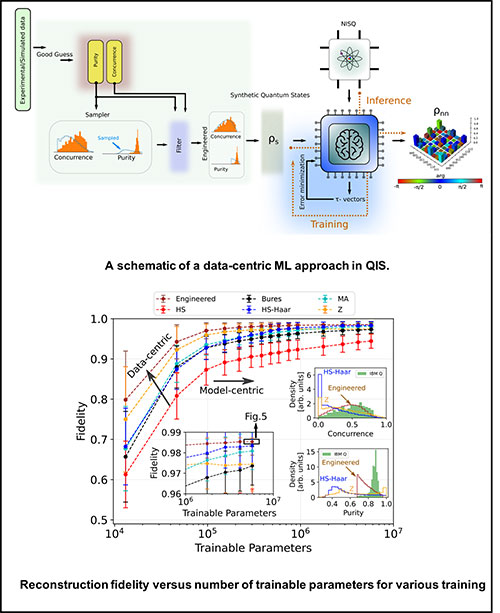
Authors: S. Lohani, J.M. Lukens, R.T. Glasser, T.A. Searles, B.T. Kirby
arXiv: 2201.09134.
Work was performed at University of Illinois Chicago (UIC), IL
Accomplishment
We propose a series of data-centric heuristics for improving the performance of machine learning systems when applied to problems in quantum information science. In particular, we consider how systematic engineering of training sets can significantly enhance the accuracy of pre-trained neural networks used for quantum state reconstruction without altering the underlying architecture.
Significance and Impact
Datacentric methods—leaving the system's architecture unchanged—endeavor to improve system performance by using enhanced data sets. Given the relative maturity and availability of machine learning (ML) models and systems, and how similarly many state-of-the-art models perform, datacentric techniques represent an undervalued opportunity to boost system performance.
Details
- We generated test sets using a noisy intermediate-scale quantum (NISQ) device – IBM Q computer.
- We compared the effectiveness of our distribution-engineering approach to other standard methods for generating data sets, including those capable of incorporating some amount of prior knowledge such as mean purity.
- We showed that false correlation causes our state reconstruction system to misclassify pure separable states as entangled, having only ever seen pure states that are entangled.
- We demonstrated that surprisingly few counterexamples need to be added to the training set to remedy this issue. Hence, it is prudent to include several states of every possible classification in any given data set.
- We found that, given the heterogeneity between the number of free-variables in pure and mixed states, it is not always optimal to endeavor to generate training sets that exactly match the distribution of an experimental scenario in the first place.
Extensible Circuit-QED Architecture via Amplitude- and Frequency-Variable Microwaves
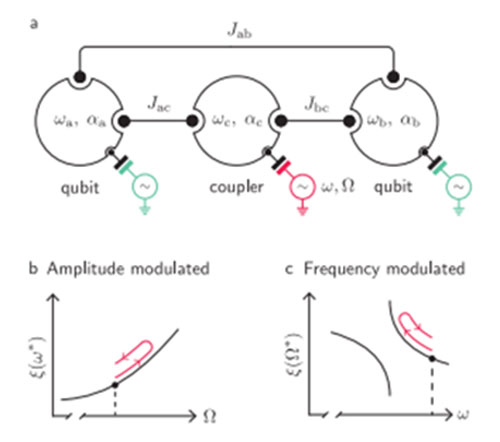
FIG a:schematic of the two-qubit-coupler architecture. Each circuit mode is represented by a Kerr-nonlinear oscillator (KNO) of frequency ωµ and anharmonicity αµ, with µ = a, b for the qubits and µ = c for the coupler. Jµν represents the coupling between modes µ and ν. T
Reference: arXiv 2204.08098
Authors: A. Di Paolo, C. Leroux, T. Hazard, K. Serniak, S. Gustavsson, A. Blais, W. Oliver.
Work was performed at Massachusetts Institute of Technology, Cambridge, MA; Université de Sherbrooke, Québec, Canadian Institute for Advanced Research, Ontario.
Accomplishment
We introduce a circuit-QED architecture combining fixed-frequency qubits and microwave-driven couplers analyzed in a driven frame.
Significance and Impact
In the appropriate frame, the drive parameters appear as tunable knobs enabling selective two-qubit coupling and coherent-error suppression.
Details
- We introduce a set of controlled-phase gates based on drive-amplitude and drive-frequency modulation. We develop a theoretical framework based on Floquet theory to model microwave-activated interactions with time-dependent drive parameters, which we also use for pulse shaping. We perform numerical simulations of the gate fidelity for realistic circuit parameters and discuss the impact of drive-induced decoherence.
- We estimate average gate fidelities beyond 99.9% for all-microwave controlled-phase operations with gate times in the range 50−120 ns. These two-qubit gates can operate over a large drive-frequency bandwidth and in a broad range of circuit parameters, thereby improving extensibility. We address the frequency allocation problem for this architecture using perturbation theory, demonstrating that qubit, coupler and drive frequencies can be chosen such that undesired static and driven interactions remain bounded in a multi-qubit device. Our numerical methods are useful for describing the time-evolution of driven systems in the adiabatic limit and are applicable to a wide variety of circuit-QED setups.
Learning Noise via Dynamical Decoupling of Entangled Qubits
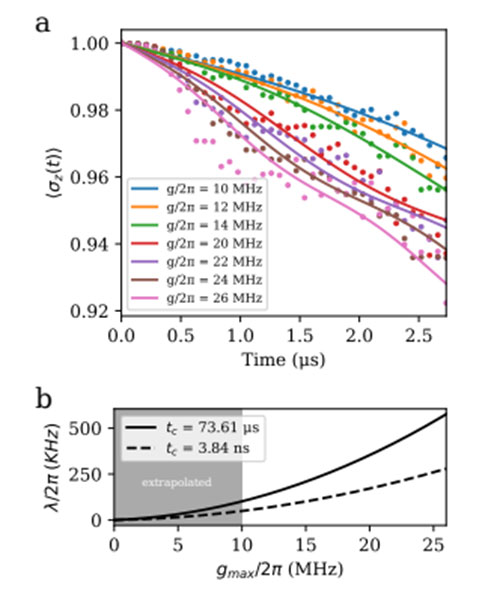
FIG.: Extracting the scaling of telegraph noise amplitude. a) Experimental data (dots) vs fit model (lines) for n = 1 CPMG sequences at various values of gmax. The fit value of Tφ is approximately 90 µs, which is a reasonable result for this device. b ) The extracted noise amplitude λ(g) for the two fluctuators. Note that the same function χΦ was used for both fluctuators; the g-noise amplitudes were only allowed to differ by an overall scale.
Reference arXiv 2201.11173
Authors T. McCourt, C. Neill, K. Lee, C. Quintana, Y. Chen, J. Kelly,
- N. Smelyanskiy, M. I. Dykman, A. Korotkov, I. L. Chuang, A. G. Petukhov
Work was performed at Massachusetts Institute of Technology, Cambridge, MA; Google AI, Santa Barbara, CA; Michigan State University, East Lansing, MI
Accomplishment
In our superconducting system comprised of Transmon qubits with tunable couplers, we observe noise that is consistent with flux fluctuations in the coupler that simultaneously affects both qubits and induces noise in their entangling parameter.
Significance and Impact
The effect of this noise on the qubits is very different from the well-studied single-qubit dephasing. Additionally, steps are observed in the decoupled signals, implying the presence of non-Gaussian noise.
Details
- Noise in entangled quantum systems is difficult to characterize due to many-body effects involving multiple degrees of freedom.
- This noise poses a challenge to quantum computing, where two-qubit gate performance is critical.
- Here, we develop and apply multi-qubit dynamical decoupling sequences that characterize noise that occurs during two-qubit gates.
Proposal for Generating Complex Microwave Graph States Using Superconducting Circuits
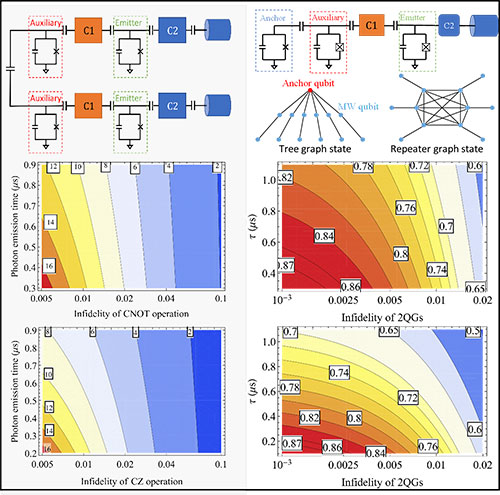
FIG.: Generation circuits (top panels) and the MW state quality for fixed frequency (middle panels) and tunable frequency (bottom panels). For MW 2D cluster states (left column), we find the number of photons with fidelity 0.8.
Authors: Chenxu Liu, Edwin Barnes, Sophia Economou
arXiv: 2201.00836
Accomplishment
We developed a protocol for the generation of microwave resource states. These are entangled ‘graph states’ that can be emitted from superconducting circuits. We calculated the fidelity of the resulting states assuming current experimental parameters and identified the main factors that limit the performance. This work serves as a blueprint for experiments.
Significance and Impact
Microwave graph states are significant for robust quantum communication between superconducting quantum processors in a distributed architecture. The encodings we consider protect against loss.
Details
- We designed generation protocols for 2D lattice and tree-like microwave graph states with realistic experimental parameters.
- We compared the performance using fixed-frequency versus tunable-frequency transmon qubits for different photonic qubit encodings.
- For both types of transmons, a 2D graph state with size can be generated with fidelity 0.8, while the tree graph state (see left figures) can be generated with fidelity 0.87.
- We identified the main factors that limiting the state fidelities for further improving the microwave state fidelities.
Quantum Search on Noisy Intermediate-Scale Quantum Devices
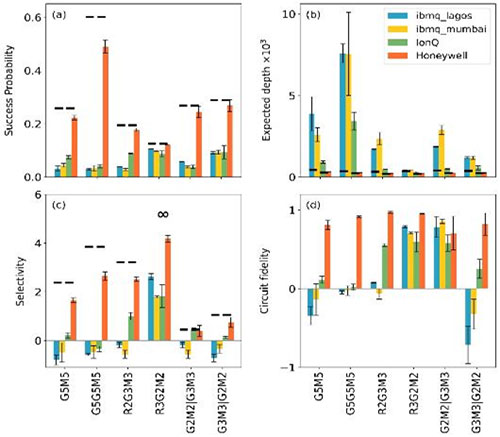
FIG.: We benchmark different implementations of five-qubit search circuits on IBM quantum, IonQ, and Honeywell quantum devices. The benchmark metrics include (a) the success probability; (b) expected depth; (c) selectivity; and (d) circuit fidelity.
Authors: Kun Zhang, Kwangmin Yu, Vladimir Korepin
arXiv: 2202.00122.
Accomplishment
We benchmarked different realizations of five-qubit quantum search algorithms on noisy intermediate-scale (NISQ) devices, including IBMQ, IonQ, and Honeywell quantum devices. We designed and implemented optimized quantum search circuits, which outperformed Grover’s algorithm. We report the highest success probability of the five-qubit search algorithm compared to previous works. We modified quantum search and made it error resistant.
Significance and Impact
The state-of-art NISQ devices can successfully run the five-qubit quantum search algorithm. Different quantum processors, with different levels of errors, have different optimal ways to realize the quantum search algorithms. To maximize the power of NISQ computers, designing error-aware algorithms is necessary.
Details
- We applied the partial diffusion operator to optimize the depth of quantum search algorithm.
- We designed the divide-and-conquer quantum search circuit in order to prevent error accumulation on NISQ devices.
QuClassi: A Hybrid Deep Neural Network Architecture Based on Quantum State Fidelity
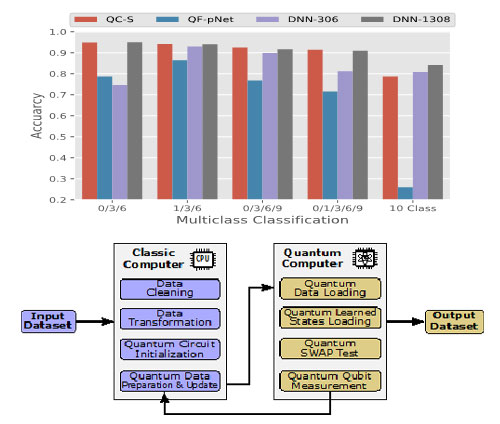
FIG.: QuClassi system architecture (quantum-classical hybrid design through variational method) and performance on multiclass classification (comparing QuClassi (QC) to QuantumFlow (QF-pNet, Jiang et al., Natural Communications, 2021) and Deep Neural networks) on the MNIST dataset.
Authors: S. Stein, B. Baheri, D. Chen, Y. Mao, Q. Guan, C. Ding and A. Li.
Accomplishment
Propose a new quantum neural network (QNN) model using quantum state-based fidelity as a loss metric for optimization, showing improved performance than existing approaches on multiclass classification tasks.
Significance and Impact
Providing a performant loss function for stable QNN training on binary and multiclass classification. Achieves comparable accuracy performance to classical deep neural network (DNNs) with 97% fewer parameters.
Details
- System design through classical data qubitization, RY/RZ/CRY/CRZ based parameterized QNN layers, and state fidelity-based cost function for gradient descent optimization
- Achieved up to 53.8% on binary and 203% on multiclass classification accuracy improvement over Tensorflow-Quantum (Google) and QuantumFlow (Jiang et al., 2021) using MNIST and Iris datasets
- Using simulators for training QuClassi models but performing inference evaluations on IBMQ superconducting machines and IonQ trapped-ion QPU.
2022-20710 | INT/EXT | Newsroom