Member Spotlight – University of Illinois Chicago (UIC)
Meet Thomas Searles, Principal Investigator at UIC, and Sanjaya Lohani, postdoc at UIC, and read about their research, "Datacentric Machine Learning in Quantum Information Science."
July 12, 2022
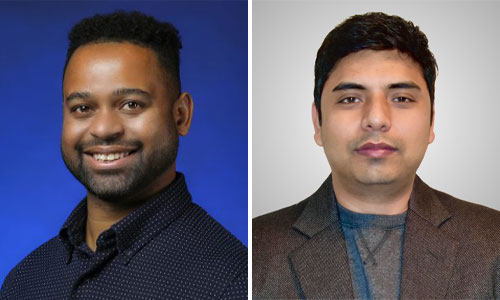
Thomas Searles and Sanjaya Lohani
Accomplishment
We propose a series of datacentric heuristics for improving the performance of machine learning (ML) systems when applied to problems in quantum information science. In particular, we consider how systematic engineering of training sets can significantly enhance the accuracy of pre-trained neural networks used for quantum state reconstruction without altering the underlying architecture.
Significance and Impact
Datacentric methods—leaving the system's architecture unchanged—endeavor to improve system performance by using enhanced data sets. Given the relative maturity and availability of ML models and systems, and how similarly many state-of-the-art models perform, datacentric techniques represent an undervalued opportunity to boost system performance.
Details
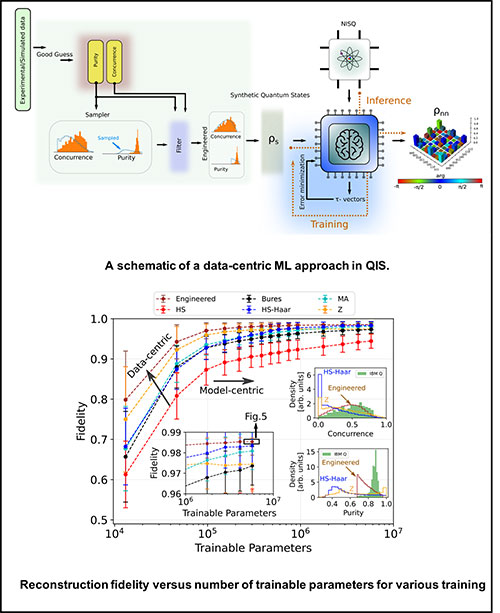
- We generated test sets using a noisy intermediate-scale quantum (NISQ) device – IBM Q computer.
- We compared the effectiveness of our distribution-engineering approach to other standard methods for generating data sets, including those capable of incorporating some amount of prior knowledge such as mean purity.
- We showed that false correlation causes our state reconstruction system to misclassify pure separable states as entangled, having only ever seen pure states that are entangled.
- We demonstrated that surprisingly few counterexamples need to be added to the training set to remedy this issue. Hence, it is prudent to include several states of every possible classification in any given data set.
- We found that, given the heterogeneity between the number of free-variables in pure and mixed states, it is not always optimal to endeavor to generate training sets that exactly match the distribution of an experimental scenario in the first place.
Authors: Lohani, S., Lukens, J. M., Glasser, R. T., Searles, T. A., & Kirby, B. T. (2022).
arXiv: 2201.09134v1. Data-Centric Machine Learning in Quantum Information Science.
Work was performed at: University of Illinois Chicago (UIC), IL
2022-20715 | INT/EXT | Newsroom