Machine Learning Deduces Material Structure from X-ray Spectra
May 29, 2020
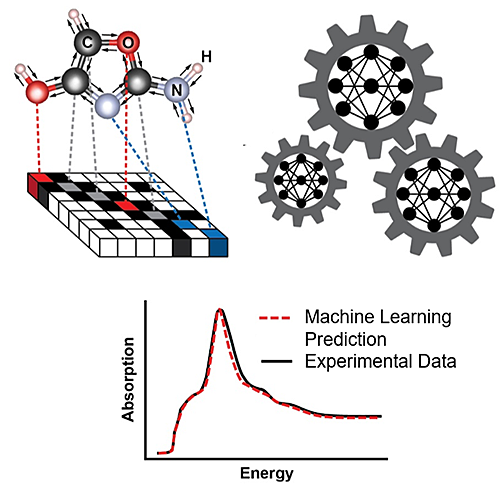
An illustration of a graph-based machine-learning model predicting x-ray absorption spectra. The molecular structure is mapped onto a molecular graph (left), which is processed by a message passing neural network model (right) that accurately predicts the spectrum (bottom).
What is the scientific achievement?
A collaborative team from Brookhaven National Laboratory and Columbia University showed that machine learning models can be trained to understand and quantitatively predict relationships between the structure of molecular systems and their x-ray absorption spectra.
Why does this achievement matter?
Unravelling the relationship between the spectral functions of materials and the underlying material structure that leads to these functions is a fundamental challenge in materials science. This study highlights the significant potential for machine learning to extract such key information from x-ray spectroscopy, which can be used for autonomous and machine-guided experimentation.
What are the details?
Simulations of excited-state properties—such as those that determine measured x-ray absorption spectra—are often computationally expensive and therefore unsuitable for high-throughput modeling. In a proof-of-principle demonstration, we show that graph-based neural networks can be used to predict the x-ray absorption near-edge structure spectra of molecules to quantitative accuracy. Specifically, the predicted spectra reproduce nearly all prominent peaks, with 90% of the predicted peak locations within 1 eV of the ground truth. Besides its own utility in spectral analysis and structural inference, our method can be combined with structure search algorithms to enable high-throughput spectrum sampling of the vast material configuration space, opening up new pathways to material design and discovery.
CFN Capabilities
The CFN Theory and Computation facility was used to simulate x-ray absorption spectra and train machine learning models.
Publication Reference
Matthew R. Carbone, Mehmet Topsakal, Deyu Lu, and Shinjae Yoo, Machine-Learning X-Ray Absorption Spectra to Quantitative Accuracy, Phys. Rev. Lett. 124, 156401 (2020).
DOI: https://doi.org/10.1103/PhysRevLett.124.156401
https://journals.aps.org/prl/abstract/10.1103/PhysRevLett.124.156401
OSTI: https://www.osti.gov/biblio/1615592-machine-learning-ray-absorption-spectra-quantitative-accuracy
Acknowledgement of Support
M.R.C. acknowledges support from the U.S. Department of Energy through the Computational Sciences Graduate Fellowship (DOE CSGF) under Grant No. DE-FG02-97ER25308. This research used resources of the Center for Functional Nanomaterials, which is a U.S. DOE Office of Science Facility, and the Scientific Data and Computing Center, a component of the Computational Science Initiative, at Brookhaven National Laboratory under Contract No. DE-SC0012704.
2020-17313 | INT/EXT | Newsroom