AI Discovers New Nanostructures Using X-rays
April 30, 2023
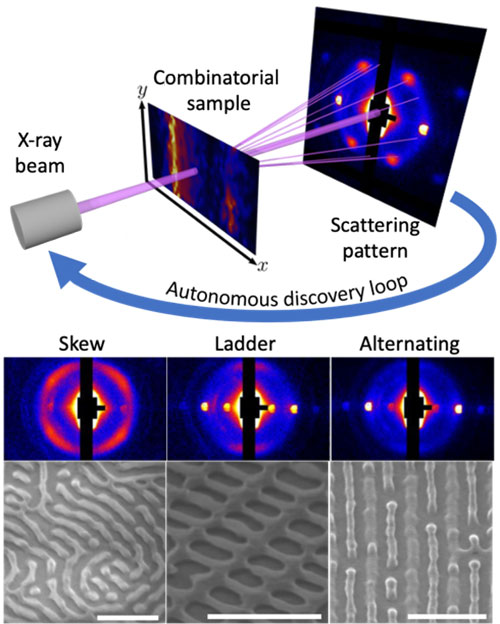
Autonomous synchrotron X-ray scattering measurements on a combinatorial self-assembled library (top) uncover 3D skew, ladder, and alternating line nanostructures.
Scientific Achievement
A CFN-led team directed a blend of two self-assembling polymers to form a large library of nanostructures. Microfocused X-ray scattering and machine learning were employed to efficiently explore the library, discovering a nanoscale “ladder” and other previously unknown 3D nanomorphologies.
Significance and Impact
This work shows how combinatorial methods, coupled with autonomous, “self-driving” characterization, accelerates the discovery of self-assembled nanostructures, which have potential applications in microelectronics, membranes, and catalysis.
Research Details
Directed self-assembly is a powerful approach to create complex nanostructure arrays and patterns by using templates to guide the self-organization of nanomaterials. Customizing these patterns promises transformative advances for manufacturing separation membranes, catalysts, and next generation nanoelectronics. However, this entails a detailed understanding of how self-assembled morphologies emerge in response to variations in nanomaterial composition, topology, and external constraints. Manual, Edisonian approaches to research are poorly matched to this high-dimensional problem, leading to incremental progress.
Previously, CFN researchers have shown that the modulating line width and period of a chemical grating template enables localized selection between self-assembled hexagonal dot or unidirectional line patterns in an overlying diblock copolymer blend. To investigate this system further, a combinatorial template with a 5× larger period range was fabricated, and Gaussian process regression (a form of machine learning) was used to autonomously guide systematic characterization of the assembled nanostructures by synchrotron small-angle X-ray scattering. This combination reduced the time required to model the system assembly behavior from more than a month (e.g., by manual electron microscopy) to several hours. In the process, completely new morphologies were discovered, including a bilayer, where top layer domains orient at a skew angle with respect to the template; alternating cylinder- and lamella-like nanostructures; and a unique “ladder” nanostructure with rectangular symmetry not commonly observed in polymer self-assembly. Coarse grained molecular dynamics simulations show that these nanostructures assemble as a consequence of localized enrichment and redistribution of polymer chains in the blend as directed by the chemical grating.
- CFN Nanofabrication and Materials Synthesis & Characterization Facilities were used to make and image the combinatorial library.
- X-ray scattering measurements performed at the Soft Matter Interfaces (SMI) beamline, operated in partnership between NSLS-II and CFN, and were guided by Lawrence Berkley National Laboratory’s Center for Advanced Mathematics for Energy Research Applications (CAMERA) gpCAM software.
- Molecular dynamic simulations performed at the BNL Computational Sciences Initiative supported the proposed assembly mechanism.
CFN Capabilities: CFN Nanofabrication and Materials Synthesis & Characterization Facilities were used to synthesize and image the combinatorial library.
Publication Reference
Doerk G.S., Stein A., Bae S., Noack M.M., Fukuto M., Yager K.G. “Autonomous discovery of emergent morphologies in directed self-assembly of block copolymer blends.” Science Advances 9, 2 (2023).
DOI: 10.1126/sciadv.add3687 (https://doi.org/10.1126/sciadv.add3687)
OSTI: www.osti.gov/biblio/1922720
Brookhaven Newsroom: AI Discovers New Nanostructures
Acknowledgment of Support
This research used the Materials Synthesis and Characterization Facility and the Nanofabrication Facility at the Center for Functional Nanomaterials (CFN), the SMI end station at the NSLS-II, and the Scientific Data and Computing Center, a component of the Computational Science Initiative, which are U.S. Department of Energy Office of Science User Facilities, at Brookhaven National Laboratory under contract no. DE-SC0012704. The work on gpCAM was funded through the Center for Advanced Mathematics for Energy Research Applications (CAMERA), which is jointly funded by the Advanced Scientific Computing Research (ASCR) and Basic Energy Sciences (BES) within the Department of Energy Office of Science, under contract no. DE-AC02-05CH11231. We thank G. Freychet and M. Zhernenkov for assistance at the SMI beamline.
2023-21263 | INT/EXT | Newsroom