AI Deduces Material Structure From Complex X-Ray Spectra
November 16, 2023
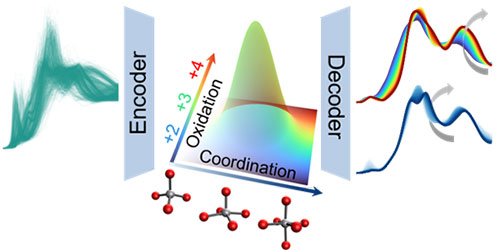
Illustration showing how the generative model (encoder) disentangles coordination numbers and oxidation states (each axis represents a target property) by performing dimension reduction in a controlled way.
Scientific Achievement
CFN researchers developed and demonstrated a new, semi-supervised machine learning model for discovering structure-spectrum relationships in x-ray absorption near-edge structure (XANES) spectra.
Significance and Impact
The decoupling of intertwined spectral contributions from multiple structural characteristics is ideal for interpreting complex scientific data and could be extended to other kinds of spectroscopic data.
Research Details
The discovery of new materials relies on the interpretation of diverse measurements, such as spectral responses. The relationships between spectral signatures and material structures are often hidden in the complex data. For example, X-ray absorption near edge structure (XANES) is sensitive to many aspects of local atomic structure and chemical characteristics. While some trends have been identified through physical arguments and empirical studies, for example, the impact of oxidation state and coordination number—and more extensive mapping of the relationship between other structure motifs and trends in the spectral features—has been elusive. In this work, we overcome these challenges with a new machine learning framework: rank-constrained adversarial autoencoders.
Adversarial autoencoders are generative models. For the XANES problem specifically, the model results in the mapping of a large database of spectra to a reduced dimensional latent space. With that mapping achieved, each point in the latent space corresponds to a possible spectrum, allowing continuous changes in spectral features to be studied over pathways in the latent space. However, the latent space variables themselves typically do not have any direct, physical meaning. To address the need for a physically interpretable latent space, we developed a novel constraint inspired by the rank correlation coefficient. Implementation of this constraint drives each dimension of the latent space to represent a target property, e.g., oxidation state of the target transition metal ion or its local coordination number, or other local structure characteristics in the target transition metal oxide crystals considered in this study.
The figure illustrates a disentangled latent space with coordination number and oxidation state: The X-axis changes the coordination number only (represented by opacity), and the Y-axis changes the oxidation state only (represented by color). The illustration shows two dimensions of the latent space. In the full study, six structure descriptors were disentangled.
The decoder was then utilized to reconstruct how the spectrum shape responds to the change of a structure descriptor, i.e., an individual dimension of the latent space. The algorithm not only reproduces known trends in the literature but also reveals unintuitive ones that are visually indiscernible in large datasets; for example, the change of main peak intensity with the change of coordination number in the second coordination shell. This new method will apply generally to other types of spectroscopies and more broadly to physical data where the correlations between source and signal are challenging to extract.
- An adversarial autoencoder was augmented with a rank constraint (RankAAE) and provided a robust and quantitative measure of the structure-spectrum relationships
- The ~57,000 simulated XANES spectra used in this work span eight first-row transition metal oxide families
- The CFN Theory and Computation Facility was used for building spectral databases and training machine learning models
Publication Reference
Liang Z., Carbone M.R., Chen W., Meng F., Stavitski E., Lu D.*, Hybertsen M.S.*, Qu X.* “Decoding structure-spectrum relationships with physically organized latent spaces.” Phys. Rev. Mater. 7, 5 (2023).
DOI: 10.1103/PhysRevMaterials.7.053802
OSTI: https://www.osti.gov/biblio/1986051
Acknowledgment of Support
This work is supported by the U.S. Department of Energy, Office of Science, Office Basic Energy Sciences, under Award No. FWP PS-030. This paper also used the Theory and Computation facility of the Center for Functional Nanomaterials, which is a U.S. Department of Energy Office of Science User Facility, at Brookhaven National Laboratory, under Contract No. DE-SC0012704.
2023-21661 | INT/EXT | Newsroom